
Is Gender-Neutral AI the Correct Solution to Gender Bias? Using Speech-Based Conversational Agents
Abstract
Background There is an issue that AI agents learn many human behaviors and values, and among them, they also learn the bias of human society. Gender bias, a significant global problem, has penetrated the domain of artificial intelligence (AI). Since AI agents are human digital assistants, it is possible to confirm gender bias in considering several AI agents, such as speech-based conversational agents, as “female.” While gender-neutral AI agents are considered the only solution, there are concerns that they could backfire on human-AI interactions. Therefore, we investigated whether interactions with gender-neutral agents are effective when compared to the expectant gender (the gender that users expect) from AI agents.
Methods We selected a “speech-based conversational agent” as a research tool that allows users to use it closely in their daily lives and intuitively judge gender. We conducted two study courses. First, we investigate the current gender status of AI agents (speech-based conversational agents). Participants who closely used gender-biased agents confirmed which voice tone and color gender they were expecting. Moreover, we checked what gender the participants expected for each task and performance experience. Second, we tested the usability of agents to which gender-neutral voices were applied. We checked how participants evaluate agents with four versions of neutral voices in terms of preference, stability, and satisfaction.
Results The first study confirmed that users perceived speech-based conversational agents as roles to perform simple tasks such as music or weather information retrieval. Moreover, participants consistently expected that a “female” would perform this role well on the side of task and experiences of task performance. The second study confirmed that participants do not prefer the gender-neutral voice of “G” because their identity is challenging to grasp. In addition, participants evaluated that some versions of “G” did not show human-like features. Thus, they did not feel stable. Finally, participants did not feel sufficient satisfaction because they did not prefer all versions of “G” and felt stable in some versions of “G.” Therefore, the participants underestimated the usability of the speech-based conversational gender-neutral agent.
Conclusions This research shows a great possibility that ignoring the expectant gender and applying gender-neutral will hinder the usability of AI agents. In addition, gender-neutral can instead be a trigger that reminds the user of the expectant gender. Therefore, we suggest that it should not be divided into human gender concepts but rather move toward genderless design that encompasses diversity.
Keywords:
Human-Robot Interaction, Conversational Agent, Gender, Gender-Neutral, Voice Gender, Human-AI Interaction1. Introduction
Recently, there have been ethical concerns and issues about Artificial Intelligence (AI) learning “Human bias.” Gender issues are a big problem in human society, and discussions about them are accelerating in the AI field. In particular, as digital assistants become more and more similar to human characteristics as technology advances (Carpenter et al., 2009), there is a “Gender bias” in which these tools (e.g., AI) that make humans convenient are gendered as “Female.” People consider the role of digital assistants as a female task. Artificial intelligence reproduces the harmful gender bias in human society (Bajorek, 2019; Costa & Ribas, 2019).
This issue of gender bias can be confirmed in detail through Speech-based conversational agents (Jacob, 2018), which have become an essential tool in daily life due to the steady increase in use. Voice recognition assistant services such as Siri were criticized for gender bias in the early days, as only the service provided female voices. Since then, it has started to provide female or male voices with various tones and colors, and recently, the world's first voice assistant service called “Project Q” has been launched to raise social awareness of this gender stereotype (Copenhagen Pride, n.d.). The voice of ‘Project Q’ provides a “Gender-neutral” voice that does not belong to either male or female (Carpenter, 2019).
In order to bring awareness of the gender bias that AI is learning, it is used as “Genderless” or “Gender-Neutral.” Genderless means encompassing diversity, unlike traditional gender concepts such as male or female. However, it should be used with a gender-neutral meaning between males and females. Therefore, forcibly creating “Gender-Neutral” is mentioned as a potential solution that AI does not learn human gender bias (West, Kraut & Chew, 2019; Barclay, 2019). However, users of these solutions have different opinions from expectations. So, it is necessary to consider whether AI, which applies gender-neutral, solves the problem of learning human gender bias and positively affects Human-AI interaction (HAII). In other words, through this research, we would like to check how gender-neutral AI, which has ambiguous gender distinction, affects real users and discuss ways to solve the gender bias issues in AI fields. We define two research questions as follows.
• RQ1. Is the gender that users expected for AI agents to be close to “Female”?
• RQ2. Compared to expectant gender for AI agents, do users think the usability of gender-neutral AI agents is good?
In this research, we selected AI agents that meet the following two conditions as research tools. First, it should be an AI agent that is frequently used in everyday life to collect user experience data. Second, it is possible to determine the gender of the agent intuitively. Speech-based conversational agents correspond to these two conditions. Robertson (2010) defines robot gender as attributing gender to robot platforms through voice. AI speakers and voice assistant services, which have high real-life usage and are used as research tools in various AI gender studies, are suitable for studying this issue (Robertson, 2010).
We want to determine the user's expectant gender in interaction with the speech-based conversational agent. In addition, we would like to see how the user evaluates interactions with neutral agents. Therefore, developing a gender-neutral AI agent is the next step in designing AI without gender bias. These findings are not limited to speech-based conversational agents but expect discussions on the actual means of the “Genderless” of AI.
2. Related work
Humans achieve smooth interaction by perceiving the identity of other people only when their gender is identified according to the agreed criteria of gender intelligence (Carpenter, 2019; Cambre & Kulkarni, 2019; Butler, 1990). In the same context, Jackson et al. (2020) stated that gender plays an essential role in recognizing and performing the norms of linguistic politeness in the interaction process. Based on this, gendered AI (Carpenter et al., 2009), an element that characterizes humans, can make one-way users feel friendly and expect high usability and accessibility. Therefore, deep consideration of gender in AI is an important and essential design area for effective human-AI interaction.
The gendering of AI is not as simple as assigning a gender. If AI is simply distinguished between male and female like human notions, users interact with different attitudes according to gender stereotypes, such as roles and behavior patterns that fit each gender (Reaves & Nass, 1996). It is common to assign the “female” gender to practical AI agents. According to Mitchell et al. (2011), it was argued that AI agents should be represented by female voices to reflect these social needs because the female gender is preferred. Similarly, Søndergaard and Hansen (2018) have revealed that the selling point of AI agents (i.e., conversational agent), which mainly provides female voices, is that agents are always available when the user needs assistance to do chores. These results commonly say that since female is a highly preferred gender, it makes users feel friendly to the agent and expect a positive effect that they can easily access it. However, the reason why the gender of “female” is naturally recalled in the role of digital assistants, such as “Voice assistants,” is that females played a similar role in human society. In other words, it originated from gender bias.
Due to simple preferences or perhaps gender bias that human society has learned for a long time, the current AI gendering implies the possibility that AI can quickly learn the cultural gender bias that human society had and, conversely, transmit bias back to humans (Song-Nichols & Young, 2020). In addition, if more and more AI agents become common and essential tools in human life, human existing gender bias will be strengthened (Rea, Wang, & Young, 2015). Studies by Reich-Stiebert and Eyssel (2017) and Zhao et al. (2017) showed that gender bias has a significant impact on user task performance; thus, repeatedly trained AI agents can worsen the existing bias. The status of AI gendering reflects the existing gender bias in human society. The issue continues whether it is right to apply gender to AI in a way that divides gender dichotomously and gives roles. Therefore, the AI field needs various discussions on AI gendering design.
In the AI domain, speech-based conversational agents are commonly used in everyday life. The development of voice assistants was predicted in the 2010s, and various integrated functions of speech-based AI are expected in the 2020s (Schwartz, 2019). Owing to the COVID-19 pandemic, as more time is spent at home, voice assistant owners increasingly use the device, and further integration with other products is being promoted (Schwartz, 2019; National Public Media, 2020). Consequently, speech-based conversational agents are becoming more common, and their influence has become more potent. However, they tend to be discriminating in terms of race and gender, thereby raising the critical issue that AI agents have learned considerable human bias (Adams & Lloydáin, 2019).
In order to solve the existing gender bias in speech-based conversational agents, a study on AI agents with neutral voices, such as ‘Project Q’ co-produced by Copenhagen Pride and four other companies, has recently emerged (Carpenter, 2019). However, users who have used these agents directly answered that they felt an Uncanny valley (Mori, 1970), a point of discomfort when robots were too similar to humans. Gender-neutral voices such as ‘Project Q’ are becoming sophisticated enough to feel like humans somewhere due to technology, but gender is not distinguished, such as male or female. Furthermore, since the human characteristics of recognizing identity are minimized except for the voice, users can think negatively of the agent (Carpenter, 2019; Cambre & Kulkarni, 2019; Butler, 1990). In other words, there are many opinions that the speech-based conversational agent of neutral voice, which is evaluated as Uncanny Valley (Mori, 1970), can cause issues in accessibility or usability to the agent rather than solving gender bias. Therefore, experts expect the AI agent that is “Gender-neutral” to break down human gendering customs. However, it is necessary to consider whether AI development is in the right direction with this gender-neutral.
3. Research design
3. 1. Research terms
To design a clear and consistent study, we would like to define the terms used throughout the study. In this study, we used and interpreted gender terms summarized by Butler (1990) and Hines (2018). Based on the existing gender concept (Gender dichotomy) and the recently emerging gender concept (Gender spectrum) in Figure 1, the research terms mainly used in this study are summarized as follows.
• Gender: A term that refers to the overall characteristics divided into social and cultural gender, masculinity, and femininity.
• Gender Dichotomy: The concept of dividing gender into male and female. Recently, the concept of neutrality has been inserted to include gender diversity. In this study, this concept is referred to as an existing gender stereotype. (Figure 1a)
•Gender Spectrum: A concept suggesting that gender includes both the characteristics of the extremes of masculinity and femininity. (Figure 1b)
• Gender Neutral: Gender in the middle of men and women, based on the concept of gender dichotomy (Figure 1a)
• Genderless: Based on the concept of the gender spectrum, an individual may be close to or include masculinity or femininity. In other words, it transcends the concept of the existing gender dichotomy and means that there are various genders within the individual (see Figure 1b)
• Gender Bias: A phenomenon in which human-like objects are perceived only by one gender based on existing gender stereotypes (the concept of gender dichotomy). This study means that human-like objects (artificial intelligence) are recognized or designed only as ‘female’.
• Expectant Gender: A term defined throughout this study to collectively refer to the gender and its characteristics that users expect from artificial intelligence, a human-like object.
3. 2. Method
This research explores the meaning of gender in human-AI interaction from a user-centered perspective and how AI gender design should be approached. We use a mixed research method, including an online survey and a Wizard of Oz test, to increase the data’s validity and present detailed analysis results and discussion points. This method is similar to the one used in the study by Behrens et al.(2018).
This study aims to explore the meaning of gender in Human-AI interaction from a user-centered perspective and consider how to approach AI gender design. Previous research has noted that it is necessary to assign gender to AI, and the influence of gendering reflects existing biases in human society. Therefore, we aim to identify the characteristics of gender bias that can be observed while using AI. To do this, we will survey to investigate what kind of Expectant Gender (the gender that users unconsciously expect) users have in the process of interacting with speech-based conversational agents, which are commonly used in real life, and what features it has. By exploring Expectant Gender, we hope to identify the current address of AI gender bias.
Second, previous research has highlighted that gender-neutral AI can be a viable solution for mitigating AI gender bias. However, concerns have been raised that using neutral AI, whose identity could be more explicit, may produce the negative side effect of being inconvenient to use rather than fulfilling its intended purpose. Therefore, it is necessary to evaluate the effectiveness of neutral AI in addressing human gender bias. In this research, we use a Wizard of Oz (WOZ) format to test the users’ evaluations of gender-neutral speech-based conversational agents. We will be able to compare this usability result with the usability of agents with the expectant gender. Through this test, we hope to provide insight into efforts to reduce gender bias in AI and consider the potential of genderless solutions.
4. Study 1: Survey of existing and expected gender voices in a conversational agent
4. 1. Study design
This survey was conducted in the following two stages to understand the gender and characteristics expected by users in speech-based conversational agents (also known as AI speakers or AI voice assistant services).
(1) We checked the usage status of existing speech-based conversational agents to find voice characteristics and gender.
(2) We conducted a survey on the gender expected by users from speech-based conversational agents by evaluating tasks and experience of task performance.
First, the overall status of speech-based conversational agents, such as devices and service types, average usage time, and main tasks, was identified. In addition, the voice of the agent was characterized by 1) tone, 2) color, and 3) appearance of gender, and investigated in detail.
The voice tone (1) was identified by dividing it into three physical representative features of sound and three types of tone. In the study of Feigen (1971), loudness and pitch denoted the physical characteristics of sound, and also speed is a factor to consider. The specificity of sound, i.e., timbre, suggests the mood of the sound, such as the attitude and emotion of the speaker, noting that it depends on the complexity (Feigen, 1971). The voice tone defined in this study refers to the physical characteristics of each sound pressure or the mood of the sound at several frequencies. As presented in Table 1, specific voice tone characteristics can be investigated through six parameters for the two significant categories of voice tone.
We defined voice color (2) as the voice characterization of the agent and voice tone. The voice color defined in this study refers to the degree of intimacy between the listener and the psychological distance (cold color) and close (warm color). Table 2 lists the specific voice color characteristics. Eight detailed items can be studied for the two significant categories of voice colors.
Voice gender (3) was inferred using the voice tone and color as defined above. In this study, we described the voice gender using gender dichotomy(Male or Female). We expected that specific descriptions can be given using the surveyed voice tone and color.
Second, we checked the gender expected by users in the voice of speech-based conversational agents. Reich-Stiebert and Eyssel (2017) investigated the voice of gender for each task to specifically describe gender bias. In the survey, six task lists were formulated for speech-based conversational agents (play and search music, search weather information, search traffic information, search the internet, receive and send phone calls, and operate TV functions). The six task lists, mainly performed by speech-based conversational agents, were formulated, and it was found that “music playback and search” was the task most performed by speech-based conversational agents. In the process of utilizing agents for the task most performed by speech-based conversational agents(music playback and search), we tried to identify the gender of the agent that users prefer, feel stable, and are satisfied with.
In the survey, we provided video data containing the process of “music playback and search” between users and agents. In this video, a user requested a task via text as shown in Figure 2. The agent who received the command was represented by the voice of ‘NAVER CLOVA Dubbing1)’ and it progressed the conversation. The female and male voices were that of “Ara Joy” and “Minsang,” respectively.
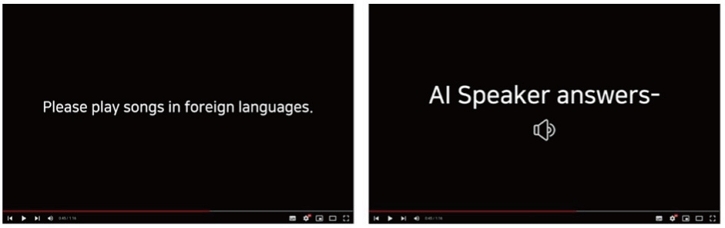
Study 1: A capture of video for second survey (Scene that user request a task via text and scene that AI Speaker response via the voice of NAVER CLOVA Dubbing from left image).
We checked significant differences in preferences and stability between genders (male/female) according to functional performance (positive/negative performance). In addition, the degree of functional performance (positive/negative performance) was investigated through satisfaction to confirm that it had a significant effect on the experience. We conducted a pre-survey to determine if the participants were correctly aware of the gender of the provided voice. Only participants who recognized the gender were used to collect the evaluation data. Table 3 compares the voices of male and female gender agents who performed the “music playback and search” task positively or negatively (2×2 within-subject design).
4. 2. Procedure
The survey consisted of four question sections, namely, the demographic information, the status of the speech-based conversational agent, the agent voice tone and color survey questions, agent voice gender expectation by task, and agent voice gender evaluation by functional performance. We created the surveys via Google Forms. Excluding demographic information questions, the survey consisted of 29 questions. The time taken for completing both surveys was approximately 10 min.
We conducted the follow-up interviews to further understand the opinions of participants who provided unusual answers. These interviews consisted of four question sections, namely, the reason for the survey answer, selection and reason for preferred/non-preferred voice in social conversations, opinions on neutral voice, and selection of expected voice for each task. The follow-up interviews were conducted over the phone; they consisted of 17 questions and took an average of 15 min.
4. 3. Participants
To efficiently record the opinions of participants and evaluation data on speech-based conversational agents, in this survey, the participation conditions were limited to the age 20 - 39 with less reluctance to technical services and devices. We divided participants into actual users who use speech-based conversational agents in their daily lives and non-users who have used agents once or twice. We believed that differences in their opinions would be and recruited real users and non-users at a 1:1 ratio. By specifying the conditions in this way, a notice was posted on personal SNS to recruit participants.
A total of 72 people, 32 men and 40 women, participated in the survey. Section 4.5 discusses the results obtained from the data of the 72 participants through significant statistical analysis. Ten participants who provided unusual answers participated in the follow-up interviews. Moreover, the minimum number of people (10) required for qualitative analysis was selected for the follow-up interviews (Creswell & Creswell, 2018).
4. 4. Analysis
This survey sample had a considerable size (n = 72) and quantitative statistical results were obtained using SPSS. We analyzed the data based on quantitative analysis; further, we performed qualitative analysis to analyze the insights. One-way ANOVA was used to compare the results with real/non-users (see Section 4.5.2). One-way variance analysis was conducted to compare whether there was a significant difference between the characteristics expected by the participants in speech-based conversational agents and the actual agent’s characteristics, and which group of participants showed a more significant difference. The results of the status level described the surveyed data in their original form (see Section 4.5.1). Also, the data collected in the survey were subjected to a t-test or frequency distribution analysis to summarize the selection frequency values and compare the results (see Sections 4.5.3 and 4.5.4). The data collected in the follow-up interviews were analyzed for the previous statistical results through qualitative analysis using the constant compatible method of ground theory (Glaser & Straus, 1967).
4. 5. Results
According to this survey, users used speech-based conversational agents present in smartphone services such as Galaxy Bixby (34.78%) and iPhone SIRI (23.91%) at least once a day (40.00%). The agents mostly performed tasks such as searching for weather information (22.08%), music playback (20.78%), and schedule management functions, such as timer (18.18%). Most participants (67.57%) answered that they were real users who used speech-based conversational agents in their daily lives, but now they have no need for agents (41.43%) and have stopped using them. Also, non-users confirmed music playback and search (30.86%) and weather information search (25.93%). In the results of these data, the participants (regardless of actual or non-user) want the speech-based conversational agents to perform simple and daily tasks such as “music” and “weather search” instead of them.
To find the answer to “What voice characteristics do users prefer in AI agents for performing a task?”, we set the focus on the voice tone and color. First, as shown in Table 4, the voice tone was investigated considering five parameters (loudness, pitch, speed, attitude, and emotional state). The results were classified according to a 6-point scale, wherein weak, low, slow, commanding, negative, and cynical tones denoted one point and strong, high, fast, convincing, positive, and optimistic denoted six points.
Participants, on average, indicated “strong (M = 3.20, SD = 0.87)” and “high (M = 3.34, SD = 0.97)” for the voice tone (n = 72) among the existing speech-based conversational agent, and the speaking speed was “difficult to see fast (M = 2.77 and SD = 0.84).” Most participants stated that the voice of the agent was “convincing (M = 3.6, SD = 1.03),” “positive (M = 4.20, SD = 1.05),” and “optimistic (M = 3.51, SD = 1.07)”; the three items demonstrated the individual difference of opinion.
Based on the voice tone of the existing agent, we examined the difference in expectations regarding the agent’s voice tone in real/non-user groups. We used one-way ANOVA to determine if a significant difference was present between the existing agent voice tone and the agent voice tone expected by real/non-users for each of the six items. Bonferroni’s post hoc test was used to check for differences. No significant difference was found in terms of strength, pitch, two attitudes, and emotions. However, there was a significant difference between the voice tone of the existing agent (M = 3.23, SD = 0.73) and that expected by actual users (M = 3.85, SD = 0.67) only in the “slow-fast” items (F(2, 52.36) = 5.05, p < .05). The users considered the “speed” at which the speech-based conversational agent delivered information and determined the necessary changes.
In addition to these results, “I do not use AI when I am in a hurry. I guess the AI agent is slow. (P7),” “The car navigation voice has become too slow and mild since its recent update. Because I have to concentrate on the road, I wish to feel a little more determined and emphasized. As shown in (P6),” It can be inferred that the speech-based conversational agent as a “conversator who needs to wait because the response speed is slow” is fixed. “It does not feel like ping pong like talking to people, so I think it is a little slow. However, this is not very reliable. (P2).” As such, it is difficult to believe that any task performed by a speech-based conversational agent, a “conversator who feels insufficient due to the slow response speed,” so we found that speech-based conversational agents find it difficult to believe that they will perform any tasks and this point should be improved.
Second, we studied the voice color considering eight elements of speech-based conversational agents (cold and hard, artificial and businesslike, intelligent, clear, calm and stable, soft and Gentle, friendly and kind, and cheerful and bright). Table 5 classifies the eight items in the form of a spectrum from the upper “cold” tag to the lower “warm” tag. We collected data through duplicate selection for eight items and determined the results.
Table 6 summarizes the ranked results, which can be expressed in the lower voice color. These lower voice color results denoted items selected by participants at least twice as many times as other upper color values. Compared to the voice color of the actual speech-based conversational agent, what participants expect is overlapping items such as “friendly and kind” and “calm and stable.” Considering only these results for agents, unlike cold voice colors, “it felt like a human feeling (P2).” It provided a warm and convenient voice color.

Study 1: Results for comparison between the actual voice color and the expectant color of speech-based conversational agents
However, the “warm” convenience voice color does not just mean “optimistic.” For the agent voice color expected by real users, “clear” and “cheerful and bright” received the highest rank. This implies that actual users want a voice color that seems to perform functions “more reliably and clearly” than the existing agent. For the agent’s voice color expected by non-users, it is like the actual agent’s voice color, but “cheerful and bright” are ranked at the top together. “I think the voice provided to the existing voice service is a bright image. I do not think I have ever felt much trust, even though it was easy to hear (P10)” and “Because the existing products or services are optimistic, I did not have much confidence. As can be seen in (P4)”; thus, it can be inferred that non-users who do not currently use the agent want the agent voice color to be “intelligent and clear.” In other words, all users expect a trustworthy agent that can provide precise answers and efficiently perform the requested tasks.
Combining the results of the expected tone and color of the speech-based conversational agent, participants displayed minimal confidence in the ability of the agent to perform a task efficiently owing to slow reaction speed. Thus, it can infer only the routine and simple tasks that it would expect to perform explicitly, such as playing/searching for music and providing weather information (see Section 4.5.1). The follow-up interviews confirmed that limited awareness and use of speech-based conversational agents are responsible for the gender assigned by users to AI agents. “I feel that a woman’s voice has a light tone, so I do not think there is any resistance to requesting such simple tasks. (P1),” and “I feel that the female voice conveys a lot better, so I keep using it for everyday tasks. After all, AI is only used to find music or weather. (P6)”; accordingly, we found that the specific gender of a “female” voice and “belief” about task performance was mentioned together.
According to the survey, users continuously used the voice of the speech-based conversational agent without changing the initially set “ female” voice (82.86%). In contrast, some participants said, “if the voice is too mechanical, it bothers me. Choose a voice that is close to what a person says. (P8),” and “When I heard the alarm, the high notes (specific to women) bothered me. (P7)” Similar to P7, there was an opinion that participants were concerned about the initial set-up “female” voice owing to the somewhat artificial characteristics of the voice (17.14%). In addition, some participants questioned the limited and consistent gender of the agent, such as “Because I only use a gentle female voice (P25).” In other words, although there is a psychological basis for the voice characteristics and gender of the speech-based conversational agents, the participants did not take any action to change the initially set “female” voice. Also, non-users also answered that they recognized speech-based conversational agents as women (81.08%), so we can infer that most users give female gender to agents.
To further check why users do not change the initially set “female” voice of the speech-based conversational agents, for each task, we investigated the expected gender of agents (Table 7). The survey helped to determine the gender of the speech-based conversational agent that performed the six tasks (music playback and search, weather information search, traffic information search, internet search, phone reception and call, and TV power).
A male and the gender indistinguishable between males and females (gender neutrality) can be compared with females by integrating into the ‘other’ gender. Consequently, we found that in all tasks except searching for traffic information for real users, more than half of real and non-users said that they expected a female voice. To determine if there is a significant difference in the “traffic information search” task for actual users with relatively large “other” gender values, an independent two-sample t-test was conducted to confirm the difference in results between actual and non-users based on tasks. No significant difference was found between the actual/non-users in all six tasks (p > .05). In other words, we confirmed that all participants generally expect a female voice of the speech-based conversational agent regardless of the task.
We investigated how the expectant gender eventually affects user experience. Participants observed the interaction between the user and the agent performing the task requested by the user through the video. Based on their experience in performing these tasks, participants evaluated each gender in terms of preference, stability, and satisfaction.
Table 8 summarizes the results of the frequency distribution analysis of three items. It can be checked that the “female” agents received a high experience of task performance for positive/negative functions. Also, regardless of the experience of task performance, participants answered that they felt more preferred and stable when using the “female” agents. The items of “satisfaction” were investigated on a 5-point scale to obtain results on the overall experience of using speech-based conversational agents. We used the paired samples test to investigate the difference in satisfaction with the experience of task performance(positive/negative). The results showed that satisfaction with experience performing positively (M = 3.80, SD = 0.82) was significantly higher than that with experience performing adverse (M = 2.70, SD = 0.88; t(39) = 6.58, p < .001). In other words, the user experience was positive when a female speech-based conversational agent performed a positive task performance.
4. 6. Study 1: Summary
From the results presented in Section 4.5, it can be concluded that the speech-based conversational agent expected “female” to be an expectant gender because intercommunication is possible at an appropriate high speed, such as human-human conversation. The answer may be the form of the user's expectation gender for RQ1's AI agent (speech-based conversational agent).
Users want human-like communication with the speech-based conversation agent of “fast” and “warm” voices (see Section 4.5.2). However, because it has not yet been implemented in this area, most speech-based conversational agents are perceived as “agents who easily handle simple tasks,” such as searching for music or weather information (see Section 4.5.1). Overall results consistently confirmed a generalized perception that “female” is suitable for performing tasks (see Sections 4.5.3 and 4.5.4); “I think it would be better for a man to think that the agent delivers information in general, but I think it is much more natural and less repulsive to be a woman if the agent explains something closely every day in the life. (P7).” Thus, through this study’s quantitative and qualitative investigation, it was confirmed that participants gave ‘female’ to AI agents (speech-based conversational agents) that help with human tasks. Through this, we confirmed the possibility that gender bias derived from humans continues in AI agents.
5. Study 2: Usability test for the gender-neutral conversational agent
5. 1. Study design
In this study, we identified how users evaluate the usability of the speech-based conversational agent with a gender-neutral voice. Participants asked agents of four gender-neutral voices (The test tool: “G”) to perform tasks and evaluated each agent’s usability (preference, stability, and satisfaction). Later, through in-depth interviews, we obtained reviews from the perspective of voice gender. In this process, we tried to check how the agent of the voice, which was gendered as neutral, affects usability.
The speech-based conversational agent with gender-neutral voice “G” was produced as the voice of “NAVER CLOVA Voice.” According to the website of Naver CLOVA Voice, they provide the hybrid form of Unit-selection and Deep Neural Network technology, providing the best synthetic voice in various domains. They provide a hybrid form of unit selection and deep neural network technology, providing the best synthetic speech in various domains. These synthetic voices in various areas are classified into contexts such as roles and occupations rather than gender. We could find “gender-neutral” voices that were not distinguished as male or female, and among these voices that included “Diversity.” As mentioned in the study by Feigen (1971), the voice tone of the test tool “G” can be classified as the most accessible pitch element to distinguish physically. We divided the gender-neutral voices of “G” into voice tones (high pitch or low pitch) and colors (warm or cold), as shown in Table 9, so that participants can intuitively evaluate different gender-neutral voices. Four versions of the gender-neutral voice of “G” with low or high pitch and cold or warm color were produced, compared, and evaluated (2×2 within-subject design).
We controlled the two features (reasonably fast response speed and precise task performance) most expected by users to evaluate the four versions of the gender-neutral agent.
First, the test tool “G” adjusted the a) response speed and b) delivery speed to maintain a moderately fast conversation pace with the participant. When a participant requested to reduce the speed at which the agent responds, a Wizard of OZ test was designed, a format in which the experimenter manipulates the agent who responds to the user without the user knowing it. Because the test evaluates participants who believe that they are testing actual speech-based conversational agent prototypes, we can expect the evaluation data to remain unchanged. After the evaluation, only participants who answered through contextual interviews, i.e., “I thought/believed that I was talking to an actual AI agent,” were used as evaluation data. Moreover, because there was a result that the delivery speed for the performance result felt somewhat slow (see Section 4.5.2), the speech speed selected for the study was adjusted slightly faster. Likewise, only participants who answered that the agent speed was “appropriate” or “not a problem” used these evaluation data.
Second, the test process was controlled as a single scenario to ensure that “G” provides more definite answers to the participant's requests. The scenario task was selected as the most preferred task (schedule management and weather and traffic information search) when performed by another gender voice, not female, in the first study. By synthesizing the three tasks, we constructed a conversational scenario between the participants and agents to check the schedule and current weather before leaving the house for an appointment and receiving recommendations for transportation and routes to the appointed place. As presented in Table 10, participants can request or respond to the agent by selecting one of the four responses in each scenario and naturally moving on to the next step to continue the request or respond to the agent's response. After the evaluation, only the participants who answered “the story flow of the scenario was natural” or “it did not interfere with achieving the real goal of the test” through contextual interviews were used as evaluation data.
Thus, for usability evaluation of speech-based conversational agents of gender-neutral voices, we produced “G,” an agent of four gender-neutral voices, adjusted in tone (high or low pitch) and color (warm or cold). In addition, we matched the “speed” that will affect usability in the non-gender testing process to the user's expectation level. Finally, we have controlled user scenarios with “tasks” that gender-neutral voices are familiar with performing from the findings of Study 1. Based on these study designs, we tried to confirm the usability of gender-neutral speech-based conversational agents in terms of 1) preference, 2) stability, and 3) satisfaction (Table 11).
5. 2. Procedure
We conducted a Wizard of OZ test using “G” with four versions of gender-neutral voice in the order of the pre-provided scenarios. Participants selected the four versions of “G” randomly; they were unaware of the purpose of evaluating a gender-neutral speech-based conversational agent. When the test was completed for each version, we studied voice keywords using eight parameters of tone items (loudness, pitch, speed, attitude (commanding-convincing, negative-positive), emotion, color, and gender, as shown in Table 12. Next, we displayed the relative position of each voice on a graph in which the tone of pitch (low-high) denoted the x-axis and the color (cold-warm) denoted the y-axis. This test was conducted through in-person meetings, and it took about 20 minutes on average.
Subsequently, we conducted follow-up interviews to obtain the comprehensive opinions of participants regarding the evaluation results and observed their behaviors and expressions. The interview comprised 21 questions, including the recognition of test tools and processes, evaluation results and reasons for observed actions, description of provided voices, reasons for voice selection and use in three aspects (preference, stability, and satisfaction), actual voice for applied to the agent and the reason, opinions on the use of the gender-neutral concept, and the test's purpose were confirmed. The interview was conducted immediately after the evaluation, and it took about 20 minutes on average.
5. 3. Participants
For this study, participants who did not experience any difficulty in using speech-based conversational agents, i.e., actual users who use these agents in everyday life, were recruited. For Study 1, we limited the age of participants to people in their 20s and 30s who were less reluctant to provide technical services and devices. In addition, overlapping participation was restricted. The conditions for participation in Study 2 were limited to subjects who did not participate in Study 1. In this study as well, overlapping participation was restricted to ensure that the evaluation results remain unaffected, including tests using “G.” Without mentioning the actual purpose of this study, participants were recruited by specifying the previous participation conditions for a “speech-based conversational agent prototype evaluation test”; these participants were also recruited from the school community and personal SNS. Finally, a total of 12 male and female participants were selected. This is the minimum number of people that can be qualitatively analyzed with the evaluation data through a usability test (Nielsen, 2012). Furthermore, it is the minimum number of people required to perform qualitative analysis with in-depth interview data (Creswell & Creswell, 2018).
5. 4. Analysis
The data collected through subsequent in-depth interviews conducted after the test were open-coded into individual events (Glaser & Strauss, 1967) or units (Lincoln & Guba, 1985) using the continuous comparative method of ground theory (Glaser & Strauss, 1967). We categorized the data several times to organize the analysis results. The qualitative results were determined by dividing them into core themes by synthesizing the categorized analyses and previous test evaluation data (see Section 5.6).
5. 5. Results
Participants commonly said, “It feels neutral overall. (P10),” and “It is not easy to distinguish between gender.” (P2), thus confirming that G is a gender-neutral AI agent, which was manufactured for this test and did not encounter any problems during evaluation. We attempted to understand the overall voice image perceived by the participants, including tone, color, and gender, for the four neutral-gender voices of G. Accordingly, a 4 min graph was used for the x-axis, divided into (left) low and (right) high pitch, and the y-axis into (left) warm and (right) cold. Each denominator was divided into 7 × 7 compartments so that the participants could express the relative difference in voice in more detail. Figure 3 shows the results of using a graph with voice tone pitch and color (warm-cold) as an axis and displays the corresponding graph denominator and the exact position as participants listen to each version of the voice of G.
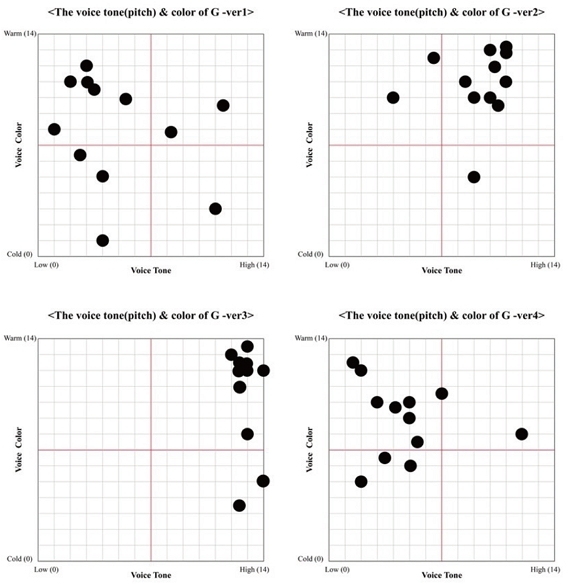
Study 2: Voice keyword for four versions of "G" (G -ver1, ver2, ver4, and ver3 clockwise from the left-top graph). The number of participants displayed on each scale is written; the larger the number, the darker the background color of the scale.
We studied voice keywords using a semantic differential scale consisting of eight parameters (loudness, pitch, speed, attitude (command-advocative, negative-positive), emotion, color, and gender). We arranged the keywords of each item in the opposite manner and checked the results using a 5-point scale at both extremes. Each scale ranged from -2 to +2, and we calculated the score for each item. The left-side items indicated negative attributes, such as weak, low, slow, imperative, pessimistic, cynical tone, and cold color; the right-side items indicated positive attributes, such as strong, high-pitched, fast, persuasive, positive, optimistic tone, and warm color. We calculated the average scores for each of the eight items. The corresponding version of the voice keywords is displayed on the number line in Figure 4.
Through Figure 4, it was difficult to grasp the tone and color patterns of the version 1, version 2, and version 4 voices of the participant's thoughts. In addition, the voice keyword scales of version 1, version 2, and version 4 voices were also close to zero, making it difficult to identify distinct features. However, we confirm that, unlike the other three versions, the tone and color patterns of version 3 speech are relatively dense in one spot. In addition, it was confirmed that the scale of the voice keyword was characterized by +12.50.
In the follow-up interview conducted to review the usage experience of the four versions of G, we attempted to investigate how the participants perceived the identity of each version. Table 13 presents a collection of adjective modifiers that express the perception of each version. The adjective “neutral” appeared at least twice for all four voices. However, there were slight differences in the adjectives of each version’s list (Table 13). Version 1 voice received the highest number of neutral expressions; however, it contained more harmful or cold color expressions than other versions. For version 2 voice, several general expressions such as “the degree to which it was relatively indistinguishable”; it is expressed like “youth boys” were used. Similarly, the version 3 voice was expressed as “young” and “cheerful.” In contrast, the version 4 voice was perceived to have a negative or colder expression than versions 2 and 3, but it was confirmed to be relatively “calm.”
In summary, participants recognized each version of G’s voice as follows: Most participants noted that Version 1 voices were “cold” and “distinguished” and answered that a voice tone and color matched the “machine.” For this reason, it was confirmed throughout the interview that participants described Version 1 voice as the most “neutral” voice. In addition, the version 3 voice goes beyond expressing a high tone and warm color, and specific characteristics such as “age” are described.
We confirm G’s usability (preference, stability, and satisfaction) with four versions of neutral voice applied through observations of the overall testing process and responses from follow-up interviews.
• Preference
Most participants answered the following when asked to select their preferred voice among the four versions of G. “It is hard to choose because it is ambiguous to distinguish them all, and it does not sound as comfortable as the existing voice. (P1)”. Conversely, we have confirmed the opinion of which of the four versions of voice would be the least preferred when using a real speech-based conversational agent.
We confirm that version 1 and version 4 are the least preferred. We received feedback regarding the version 1 voice: “It was creepy, but it felt like a neutral voice among the four. (P7)”; “Because the concepts of women and men were ambiguous, as the version 1 item felt that way, the other three voices felt relatively like boys. (P10).” Similar opinions to P10 prevailed. Participants chose Version 1 as their least preferred speech based on these opinions. We received feedback regarding the version 4 voice: “In fact, version 4 was not memorable, but I thought it might be frustrating because of the low tone. (P3)”; “Version 4 was not preferred in other respects compared to version 1. Version 1 was a creepy voice mixed with the voices of the two men and women, and Version 4 was uncomfortable to talk to. (P7)” In other words, we can infer that version 1 represents a human aspect. Also, we can infer that version 4 represents a human aspect, but no unique self-characteristics could be ascertained. As a result, in terms of “preference,” participants feel G negatively with a neutral voice and have not formed any meaningful connection or influence between the agent and the participant.
• Stability
During the testing process, participants confirmed that they would continuously use the agent’s voice when it felt “stable.” We analyzed why participants chose Version 3 and Version 2 among the four versions of G as ‘stable’ and ‘trusted’ voices through the following feedback. “It's the fastest one. I thought it was that natural because it was done quickly and adequately, as a real person said. (P8),” “It felt similar to my voice, so I felt strange and friendly right away. (P1),” “It felt like a smart lower-grade elementary school kid was talking. (P4)” Participants express natural, stable, and reliable when somewhat like the characteristics of a voice they know, such as the speed of most humans speak, my familiar voice, and the voice of an elementary school student (Table 13). In other words, participants mentioned the possibility of smooth communication with the agent only when they judged that the characteristics and conversation method of human voice were applied among versions of G with a neutral voice in terms of “stability.”
•Satisfaction
We confirmed that among the versions of G, the participants were most satisfied with the version 2 voice. Participants mentioned version 2 voices that felt like human objects due to their “clear identity” rather than “neutral that felt like a machine.” In addition, participants evaluated Version 2 Voice as “the most preferred voice (preference side)” and, at the same time, “similar to my voice (stability side).” Through this, we confirmed that the participants felt a certain degree of “satisfaction” with the voice that fully felt the previous “preference”and “stability.”
In other words, if the “preference” and “stability” are not satisfied with the neutral voice, it also means that participants have difficulty feeling “satisfaction” with the gender-neutral voice. Through the previous results, the “preference” and “stability” side, participants generally did not prefer G's neutral voice. In addition, the participants said they felt stable in G's gender-neutral voice only when it has the characteristics and conversation method of the human voice. Therefore, it is difficult to say that the participants were satisfied with the four neutral voices of G.
5. 6. Study 2: Summary
Based on the results in Section 5.5, participants recognized the four versions of G voices as ‘gender-neutral’ with different voice tones and colors. We confirmed that the participants' non-preferred version 1 and 4 voices caused rejection in the process of mutual communication. Participants mentioned that they felt stability in version 2 and 3 voices. In other words, participants do not prefer and do not feel stable with all versions of “G.” These results show that the four versions of G voice did not bring a satisfactory experience to the participants. Thus, a speech-based conversational agents voice agent with a neutral voice, such as G, can negatively affect usability, and this result is the answer to RQ2.
6. Discussion
Gendered AI reflects the bias of human society, and the application of “gender-neutrality” as a solution to this is emerging. In this study, we conducted the following two research processes through the speech-based conversational agent, an AI agent widely used in everyday life. First, we investigated what gender the user expected from the agent, and in this process, H1 was verified. Second, we checked the user's evaluation of the agent to which 'gender-neutrality' was applied and verified H2 in this process. Finally, we summarized the contents of hypothesis verification in each research process and the answers to the research questions as follows.
6. 1. Research conclusion
In Study 1, we were able to find the answer to Study Question 1. Through Study 1, we confirm that users of current speech-based conversational agents perform simple tasks mainly utilizing “female” voices. In addition, we confirmed that they wanted “female” voices in various voice tones and colors and that they expected “female” voices regardless of their experience in performing tasks or tasks.
In Study 2, we were able to find the answer to Research Question 2. To enable participants to evaluate “gender-neutral” voices consistently, we utilized four neutral voice “G”s with different tones and colors as test tools. Through study 2, we confirmed that users of speech-based conversational agents did not prefer “gender-neutral” voices with ambiguous identities and no human nature. In addition, we confirmed that users could feel stable regardless of gender if it is a voice similar to humans or a conversation method. Finally, we confirmed that the user could only be satisfied with the “gender-neutral” voice when this preference and stability were satisfied.
6. 2. Research Themes
From the two research processes, it can be inferred that the expected gender for a speech-based conversational agent was “female,” so the agent reflected human “gender bias.” It can also be inferred that “gender-neutrality” reduces usability regarding preference, stability, and satisfaction and therefore requires a different approach to mitigate “gender bias.” We want to summarize the discussion points through the following four themes.
In study 2, which evaluates the use of four neutral voice versions of G, all participants first provided answers for the keyword “gender.” “I don't know why, but I was the first one to check men and women.” Based on P10, it is evident that the participants attempted to unconsciously classify the gender of agent G. This reaffirms the results of Costa and Ribas (2019), who identified gender according to the agreed criteria of gender intelligibility for smooth interaction in the study by Butler (1990). Only gender (male or female) in dichotomy classification, which is the existing gender rule, is a significant factor, owing to which, AI agents are perceived as humans. In other words, it suggests that rejecting the expectant gender from speech-based conversational agents may lead to negative results between human and AI interactions.
By exhibiting gender distinction, the participants also presented essential rejection reactions such as “neutral voices are difficult and unfamiliar to distinguish. (P1)” and “the first neutral voice seems to be more awkward than other voices. (P8)” However, if a user has sufficient experience with AI agents, despite the ambiguity of gender distinction, the agent can be identified using factors other than gender. “First, I thought (all versions of the voices) were difficult to recognize as an object, but I thought that just the way of speaking and atmosphere, like the items on the evaluation paper, could function well enough. (P12)” Even if an AI agent is ambiguous in terms of gender, if the voice tone and voice color are evident in the tone, then users can be expected to perceive the agent as a conversation partner. Considering the long-term usage time, even if the impact of the gender of AI is small, the impact of characteristic factors such as conversation method, content and intuitive distance can help in understanding the identity of the AI.
Eight out of the 12 participants from study 2 answered that if they considered the currently used AI agents, including speech-based conversational agents, to be gender-neutral, the agents would be perceived as an object to be respected with honorifics instead of a classic woman. There was an opinion that neutral AI agents would be significantly more intelligent and reliable than before. Moreover, “if neutrality is applied, I think it will go well with the word artificial intelligence. (P4)” There was another opinion that the neutrality of the machine would be well utilized.
However, the opposition sharply opposed. Regardless of the gender applied to the AI agent, some participants unconsciously sought after feminine features or functions (expectant gender). “Unless devices such as AI speakers are dramatically developed considering high-dimensional functions in areas such as tone and speech, or even so, I do not think we can change our existing image. Rather, I think a more elite female secretary will come to mind. (P11)” In other words, applying “gender-neutral” instead raises the opinion that applying the existing “female” can be more effective for usability. However, this does not break away from AI agent design that only applies specific gender (female) but can instead continue “gender bias.” Thus, it can harm human-AI interaction by recklessly rejecting users’ expectations of the gender (Expectant Gender) of AI agents, including speech-based conversational agents.
Eleven out of the 12 participants from study 2 stated that the existing AI agents, including speech-based conversational agents, learn the bias inherent to humans. They agreed that if humans continue to use AI agents without further guidance for future development, awareness regarding gender cannot be raised and the gender bias may be intensified. However, as mentioned in Section 6.2.1, gender is a significant aspect for AI to be recognized as a conversational partner and it cannot be removed recklessly. In addition, it has been pointed out that the use of gender-neutral AI agents for reducing the gender bias may have adverse effects (refer to Section 6.2.2). How should we design to eliminate/minimize the “gender bias” of applying only one gender to AI, which is increasingly used in everyday life as an essential tool for humans?
The gender assigned to AI can be distinguished from that of humans and viewed as a third gender (yet unnamed). “I think the neutrality of the machine and humans is different. If I consider it as same area, I think the confusion will be too great. It is necessary to consider and develop differently AI gender from human gender standards. (P10)” Considering the results of this research, AI designers suggest that it is important to take measures to develop a third gender for AI that is different from the classification of human gender. As mentioned in Section 6.2.1, it is necessary to reinforce characteristic elements, such as conversation method, content and intuitive distance. Strengthening these characteristic factors implies that users can replace and minimize the time taken to consider whether the gender of an AI is female or male, thus eventually reducing the influence of the existing human gender classification on AI identity. In other words, in AI, efforts are needed to develop genderless AI that focuses on roles and contexts rather than gender boundaries from a human perspective.
What does “genderless” imply? It can be considered as the third gender, including diversity, which does not require boundaries or standards that distinguish gender(male or female). In study 2, it was difficult to distinguish the voice characterized by “young age” into male or female; most participants described it as a “neutral” voice. Users felt more comfortable and showed high preference to this voice because it seemed “relatively young,” “younger than me,”and “high and cheerful.” Similar to the results of Jackson, Williams & Smith (2020), when the voice characteristics of the user and robot match, a more favorable evaluation can be obtained. It was also found that in this case, users tend to feel more familiar with the voice tone and they believe that the voice color of the AI agent is similar to theirs. Because the use of characteristic elements such as closeness and familiarity emphasizes the identity of AI, it can be a potential future step toward developing genderless AI.
Nevertheless, it is necessary to assign gender to intelligent machines in certain situations and context. For example, the leading navigation service T-Map provides version 3 of G like voice in the child protection zone near elementary schools to minimize gender impact and emphasize the surrounding environment to the driver. Because users have high expectations for voices that match the appearance and image of the device in the case of speech-based conversational agents (Carpenter, 2019), the consideration of these “functional elements” that can be extracted by AI from the context of users can also be a prospective direction for designing genderless AI. In other words, designing functional elements that emphasize the context and roles of AI is expected to allow users to perceive AI as a conversation partner with the minimum influence on the existing dichotomous gender classification.
6. 3. Implication
This research used speech-based conversational agents to identify “gender biases” that applied AI to certain genders (female). Through the first research process, it was possible to describe in detail what gender users expect from AI agents in voice tones and colors. In addition, we quantitatively identified the expected gender in various aspects, such as the experience of performing tasks and tasks. Finally, through the secondary research process, we qualitatively confirm that applying gender neutrality can hurt Human-AI interaction in terms of preference, stability, and satisfaction.
Rather than relying on quantitative results, this study delivered them to the discussions on AI gendering through qualitative analysis. In order for users to recognize AI as a conversation partner and create good communication, the results of previous studies that gender is an essential element was reaffirmed. For this smooth human-AI communication, users have an expectant gender of “female,” and ignoring it recklessly and applying “gender-neutral” suggests that it is a dangerous direction that hinders usability. In addition, neutrality can instead be a trigger that reminds the user of the expectant gender, which is inconsistent with the background in which neutrality began to be applied to AI. We suggest focusing on the context in which AI will be utilized and what role it will play as a conversation target is necessary. Also, we suggest that it should not be divided into human gender concepts but rather move toward genderless design that encompasses diversity.
7. Limitations
This research limited research tools to speech-based conversational agents, AIs that are closely used in everyday life, for adequate experimental progress. In addition, we classify the tasks based on speech-based interactive agents for gender-related investigations. Research results from one type of AI agent are challenging to generalize across AI fields. It is necessary to select robots or AI agents as research tools in various situations such as education and healthcare, mention that participants expect the same gender(female), and see if similar evaluation results are obtained for neutral agents. Furthermore, the task of speech interactive agents, a research tool, was limited to simple task performance. Therefore, we can consider a follow-up study of gender impact through 'social conversation situations’ with AI agents. Furthermore, studies investigating the effect of gender of AI agents on people with physical or mental illness can be considered. In other words, based on this study, advanced follow-up studies considering various variables can be expected.
Korean participants were recruited to proceed with Study 1 and 2 quickly. Therefore, it is possible to consider whether the same result can be derived from participants of different nationalities of the same age group. In Study 1, the results were summarized using 72 participant data. However, more samples need to be collected to confirm the significance of the results for expected gender and its associated features. Because we used speech-based conversation agents, a commonly used research tool in everyday life, we distinguished questionnaire items by speech tone and color. However, items need to reflect in various ways the type of agent, the environment of use, and the task used to determine the expected gender. In study 2, the pitch of voice tone and voice color was used to classify the neutral speech of the research tool “G” into four types. Among the six parameters, we used only the pitch, the most physically accessible parameter, to distinguish voice tones. It is possible to consider using five other tone parameters through follow-up studies. We controlled the interaction process as a scenario to ensure that the agent's function did not affect the results. However, we can consider whether the same results will give participants more autonomy to interact with the agent. We evaluated the usability of participants in terms of preference, stability, and satisfaction and analyzed the results qualitatively. Through follow-up studies, it is necessary to analyze the number of people sufficient for quantitative analysis to investigate the relationship between preference, stability, and satisfaction and the significance of the results. It is also worthwhile to test a gender-neutral AI agent several times over a long time to observe a change in the user's perspective on gender. It is expected to increase the effectiveness of evaluation data by tracking changes in evaluation results over a long time.
8. Conclusion
It is emerging as a social issue that AI learns human behavior, values, and human bias. In particular, the gender bias issue, which is gendered only by one gender, is a problem that must be solved in AI. Recently, experts have tried to solve this problem by applying gender-neutral to AI. However, although such solutions can be identified in speech-based conversational agents, users tend to describe them as instead belonging to unpleasant valleys. Therefore, we wanted to investigate whether gender-neutral agents could replace AI designed with biased gender and be the next step in developing AI that does not learn human gender bias.
This research consists of two research processes. Before conducting the research, we used speech-based conversational agents frequently used in everyday life and can intuitively grasp gender as a research tool. In Study 1, we recruited 72 participants with experience using speech-based conversational agents and surveyed the status of the agents. Participants noted that they consider the agent to be an aid in performing simple tasks (searing for music or weather information) and expect this role to be performed by “fast-speed” and “warm”voices. It also noted that these voices are expected to be “female.” In addition, participants tended to expect “female” voices regardless of the type of task an agent could perform and to expect “female” voices in any experience of performing the task. Therefore, we could conclude that the “Expectant gender” of the participants was “female” through Study 1. In Study 2, we produced “G” with four neutral voice versions and conducted a Wizard of OZ-style usability test for “G” on 12 participants. All participants participated without knowing the real purpose of the test. For each voice, participants described the four versions of “G” voices as gender-neutral or gender boundaries ambiguous through the voice tone & color graph, voice keyword, and interviews. Participants evaluated that they did not prefer all four versions of the neutral voice and felt stable about some versions of “G.” Also, we found that participants were not satisfied with “G” because they had a low preference and did not feel stable for all versions of “G.” In addition, it was possible to obtain a result that somewhat reminded “Expectant Gender.” Therefore, we could conclude from Study 2 that participants evaluated the usability of the agent of gender-neutral voices as bad.
We can conclude from Study 1 and Study 2 that gender-neutral agents are unsuitable for replacing AI designed with biased gender (female). In other words, for smooth communication between users and AI, it was confirmed that it was difficult to violate the “Expectant gender (female).” AI gender design using existing human gender concepts (e.g., Gender dichotomy) still does not solve enough for AI to learn human bias. Therefore, we discussed developing AI-only gender concepts (Genderless) in consideration of diversity, such as the context or role in which AI is utilized.
In this research, using a speech-based conversational agent as a tool, the expectant gender was quantitatively confirmed in the AI agent. In addition, the usability of gender-neutral agents was qualitatively analyzed. In addition, we thoroughly discussed the results of each study and the direction in which AI will develop without gender bias. Future research can expect the development and guidelines of genderless AI agents considering environmental and social aspects.
Glossary
1) NAVER CLOVA, https://clova.ai/voice.
Acknowledgments
This research was supported by Basic Science Research Program (2021R1I1A4A01059550) through the National Research Foundation of Korea (NRF) funded by the Ministry of Education.
Notes
Copyright : This is an Open Access article distributed under the terms of the Creative Commons Attribution Non-Commercial License (http://creativecommons.org/licenses/by-nc/3.0/), which permits unrestricted educational and non-commercial use, provided the original work is properly cited.
References
- Kim, J. (2004). Analysis of Korean Design Study Tendency. Jounal of Korean Society of Design Research, 17(4), 159-168.
- Suh, J. (2003). Classification System on Enterprise Information System Research and Its Application (Master's thesis), Yeonsei Univesity, Seoul, Korea.
- Lee, S., & Lee, K. (1999). A Study on the Trend of Design Research Through the Journal of Korean Society of Design Studies. In Proceeding of Korean Society of Design Research (pp. 38-39). KSDS.
- Lee, J., Kang, B., Kim, S., Kim, H., & Hong, S. (1999). A Development of Design Database Structure and Database Application System applicable for Design Foundation Courses. Journal of Korean Society of Design Research, 12(4), 283-292.
- Buchanan. R. (1979). Theory of library classification. London: Bingley.
-
Buchanan, R. (2001). Design Research and the New Learning. Design Issues, 17(4), 3-23.
[https://doi.org/10.1162/07479360152681056]
- Frayling, C. (1993). Research in art and design. Royal College of Art Research Papers, 1(1), 1-5.
-
Love, T. (2000). Philosophy of design: a meta-theoretical structure for design theory. Design Studies, 21(3), 293-313.
[https://doi.org/10.1016/S0142-694X(99)00012-5]
-
Owen, C. L. (1998). Design Research: Building the Knowledge Base. Design Studies, 19(1), 9-19.
[https://doi.org/10.1016/S0142-694X(97)00030-6]
- Marcello, R., & Newton, R. (1983). A New Manual of Classification. New York: Gower Publishing.
-
Adams, R., & Loideáin, N. N. (2019). Addressing indirect discrimination and gender stereotypes in AI virtual personal assistants: the role of international human rights law. Cambridge International Law Journal, 8(2), 241-257.
[https://doi.org/10.4337/cilj.2019.02.04]
- Bajorek, J. P. (2019, May 10). Voice recognition still has significant race and gender biases. Harvard Business Review. Retrieved from https://hbr.org/2019/05/voice-recognition-still-has-significant-race-and-gender-biases.
- Barclay, K. (2019, March 18). The future of AI is genderless: Instead of giving everyone a subservient female assistant, what if our future AI helpers were designed to have no gender at all?. Fast Company. Retrieved from https://www.fastcompany.com/90320194/the-future-of-ai-is-genderless.
-
Behrens, S. I., Egsvang, A. K. K., Hansen, M., & Møllegård-Schroll, A. M. (2018). Gendered Robot Voices and Their Influence on Trust. In Companion of the 2018 ACM/IEEE International Conference on Human-Robot Interaction (pp. 63-64). Association for Computing Machinery.
[https://doi.org/10.1145/3173386.3177009]
-
Bryant, D., Borenstein, J., & Howard. A. (2020). Why Should We Gender? The Effect of Robot Gendering and Occupational Stereotypes on Human Trust and Perceived Competency. In Proceedings of the 2020 ACM/IEEE International Conference on Human-Robot Interaction (pp. 13-21). Association for Computing Machinery.
[https://doi.org/10.1145/3319502.3374778]
- Butler, J. (1990). Gender Trouble: Feminism and the Subversion of Identity. New York: Routledge.
-
Cambre, J., & Kulkarni, C. (2019). One voice fits all? Social implications and research challenges of designing voices for smart devices. In Proceedings of the ACM on human-computer interaction, 3(CSCW), 1-19. Association for Computing Machinery.
[https://doi.org/10.1145/3359325]
-
Carpenter, J. (2019). Why project Q is more than the world's first nonbinary voice for technology. Interactions, 26(6), 56-59.
[https://doi.org/10.1145/3358912]
-
Carpenter, J., Davis, J. M., Erwin-Stewart, N., Lee, T. R., Bransford, J. D., & Vye, N. (2009). Gender Representation and Humanoid Robots Designed for Domestic Use. International Journal of Social Robotics, 1(3), 261-265.
[https://doi.org/10.1007/s12369-009-0016-4]
-
Costa, P., & Ribas, R. (2019). AI becomes her: Discussing gender and artificial intelligence. Technoetic Arts a Journal of Speculative Research, 17(1), 171-193.
[https://doi.org/10.1386/tear_00014_1]
- Copenhagen Pride. (n.d.). (2019, March). Project Q: Genderless Voice. Retrieved from https://www.genderlessvoice.com/.
- Creswell, J. W., & Creswell, J. D. (2017). Research design: Qualitative, quantitative, and mixed methods approaches. Thousand Oaks, CA: SAGE Publications.
-
Feigen, L. P. (1971). Physical characteristics of sound and hearing. The American journal of cardiology, 28(2), 130-133.
[https://doi.org/10.1016/0002-9149(71)90360-2]
-
Feine, J., Gnewuch, U., Morana, S., & Maedche, A. (2019, November). Gender bias in chatbot design. In International Workshop on Chatbot Research and Design (pp. 79-93). Springer.
[https://doi.org/10.1007/978-3-030-39540-7_6]
- Straus, A. L., & Glaser, B. G. (1967). The discovery of grounded theory: Strategies for qualitative research. New York: Routledge.
- Hines, S., & Taylor, M. (2018). Is gender fluid?: a primer for the 21st century. New York: Thames & Hudson.
- International Women's Day. (2020, March). Gender and AI: Addressing bias in artificial intelligence. Retrieved from https://www.internationalwomensday.com/Missions/14458/Gender-and-AI-Addressing-bias-in-artificial-intelligence.
-
Jackson, R. B., Williams, T., & Smith, N. (2020). Exploring the Role of Gender in Perceptions of Robotic Noncompliance. In Proceedings of the 2020 ACM/IEEE International Conference on Human-Robot Interaction (pp. 559-567). Association for Computing Machinery.
[https://doi.org/10.1145/3319502.3374831]
- Jacob, S. (2018, September 20). The Future of Voice Technology And What It Means for the Enterprise. AppDynamics. Retrieved from https://www.appdynamics.com/blog/news/voice-technology-and-the-enterprise/.
-
Lincoln, Y. S., & Guba, E. G., (1985). Naturalistic Inquiry. Newbury Park, CA: Sage Publications.
[https://doi.org/10.1016/0147-1767(85)90062-8]
-
Mitchell, W. J., Ho, C. -C, Patel, H., & MacDorman, K. F. (2011). Does social desirability bias favor humans? Explicit-implicit evaluations of synthesized speech support a new HCI model of impression management. Computers in Human Behavior, 27(1), 402-412.
[https://doi.org/10.1016/j.chb.2010.09.002]
- Mori, M. (1970). The Uncanny Valley. Energy, 7(4), 33-35.
- National Public Media. (2020). The Smart Audio Report. National Public Media. Retrieved from https://www.nationalpublicmedia.com/insights/reports/smart-audio-report/.
- Nielsen, J. (2003, January 19). Recruiting Test Participants for Usability Studies. Nielsen Norman Group. Retrieved from https://www.nngroup.com/articles/recruiting-test-participants-for-usability-studies/.
-
Rea, D. J., Wang, Y., & Young, J. E. (2015, October). Check your stereotypes at the door: an analysis of gender typecasts in social human-robot interaction. In International conference on social robotics (pp. 554-563). Springer.
[https://doi.org/10.1007/978-3-319-25554-5_55]
- Reeves, B., & Nass, C. I. (1996). The media equation: How people treat computers, television, and new media like real people and places. Center for the Study of Language and Information; Cambridge University Press.
-
Reich-Stiebert, N., & Eyssel, F. (2017). (Ir)relevanceof Gender? On the Influence of Gender Stereotypes on Learning with a Robot. In Proceedings of the 2017 ACM/IEEE International Conference on Human-Robot Interaction (pp. 166-176). Association for Computing Machinery.
[https://doi.org/10.1145/2909824.3020242]
-
Robertson. J. (2010). Gendering humanoid robots: Robo-sexism in Japan. Body & Society, 16(2), 1-36.
[https://doi.org/10.1177/1357034X10364767]
- Schwartz, E. H. (2019, December 31). The Decade of Voice Assistant Revolution. Voicebot.ai. Retrieved from https://voicebot.ai/2019/12/31/the-decade-of-voice-assistant-revolution/.
-
Søndergaard, M. L., & Hansen, L. K. (2018). Intimate Futures: Staying with the Trouble of Digital Personal Assistants through Design Fiction. In Proceedings of the 2018 Designing Interactive Systems Conference (pp.869-880). Association for Computing Machinery.
[https://doi.org/10.1145/3196709.3196766]
- Song-Nichols, K., & Young, A. G. (2020). Gendered Robots Can Change Children's Gender Stereotyping. In Proceedings of the CogSci 2020 (pp. 2480-2485). Cognitive Science Society.
-
Strauss, A. L. (1987). Qualitative analysis for social scientists. Cambridge, UK: Cambridge University Press.
[https://doi.org/10.1017/CBO9780511557842]
-
Tolmeijer, S., Zierau, N., Janson, A., Wahdatehagh, J., Leimeister, J. M., Bernstein, A. (2021). Female by Default?-Exploring the Effect of Voice Assistant Gender and Pitch on Trait and Trust Attribution. In Extended Abstracts of the 2021 CHI Conference on Human Factors in Computing Systems (pp. 1-7). Association for Computing Machinery.
[https://doi.org/10.1145/3411763.3451623]
- West, M., Kraut, R., & Ei Chew, H. (2019). I'd blush if I could: closing gender divides in digital skills through education. UNESCO. https://unesdoc.unesco.org/ark:/48223/pf0000367416.page=1.
-
Zhao, J., Wang, T., Yatskar, M., Ordonez, V., Chang, K. -W. (2017) Men Also Like Shopping: Reducing Gender Bias Amplification using Corpus-level Constraints. In Proceedings of the 2017 Conference on Empirical Methods in Natural Language Processing (pp.2979-2989). Association for Computational Linguistics.
[https://doi.org/10.18653/v1/D17-1323]