
Investigating Preferential Usability on Intelligent Agent-Driven Multimodal Interaction within the Extended Reality Environment
Abstract
Background With the expansive development of artificial intelligence (AI)-driven technology and its widespread application, an advancement of the extended reality (XR) technology is gradually increasing the needs for intellectually navigating the diversified, multi-layered information in the virtual or mixed-reality space.
Methods By integrating the AI agent with the XR environments, this study explores the opportunity on how the intelligent agent-driven interface under the XR condition could interplay with participants and influence their perceptibility over the AI agent’s curated information via head mounted display (HMD). In shaping the characteristics of the AI agents that exists in the XR environments, two major types are dealt with in the research: An AI agent that “independently” exists in the virtual home space (ITA), and an AI agent “dependent” to a panel-type interface (DTA). Based upon these two agent types, multimodal interaction methods are primarily designed and prototyped in the processes of verbally and non-verbally communicating with them. Participants’ heuristic and preferential responses collected from the simulations are analyzed to figure out the suitable usability on multimodal interactions.
Results This study revealed participants’ preference for the AI agent perceived as physically existing in an XR environment, emphasizing practicality and playfulness in interactive experiences. Moreover, participants express a desire to alternatively utilize two AI agent types, highlighting the convenience of ITA’s ubiquitous activation and DTA’s responsiveness to content navigation.
Conclusions We suggest a blueprint for AI agent interaction in the XR environments and validate it through a UX experiment. Ultimately, this study aims to seek out an opportunity of optimizing the interaction methods towards the AI agent in an XR environment in a user-friendly way.
Keywords:
Human-Agent Interaction, eXtended Reality, Intelligent Agent, Natural Interaction, Artificial Intelligence1. Introduction
Hybridized with existing fields’ technologies and platforms, AI-related products and research are exponentially showcasing the future opportunities on how the AI-driven technology could disruptively change the way of interacting with surroundings. In terms of AI, its radical advancement in various extant platforms such as voice assistance or chat-bot improved the overall spectrum of perceiving information with highly user-friendly interactions (Wienrich and Latoschik, 2021). In addition, the developmental and industrial advancement of spatial computing technology regarding eXtended Reality (XR) is gradually fostering the needs and possibilities of hybridizing the AI agent with the virtual/mixed reality environment. In this respect, developing the AI agent-driven platform under the virtual reality environment necessitates users’ various direct/in-direct expression methods as an input, in order for them to navigate and browse the information on the 3 dimensionally augmented interface. Dominantly, in-direct expressions and commands via button-type controllers combined with a gyroscope sensor have been widely employed in the VR gaming industries, such as Beat-Saber®, a VR game based on slashing and swinging gestures via controller (Meta Quest, 2019).
With this controller-driven development especially in the gaming industries, expression methods towards the interface or holographic objects under the VR/MR environment expanded into direct, naturally intuitive expression methods—in other words, “Natural Interaction”. In terms of judging the cognitive range, a semantic definition of Natural Interaction can be subjective and vary by perspectives and systems (Chu and Begole, 2010). Mostly, hand or finger gestures are treated as a main communicative medium that represents the natural interaction, since the hand gesture-driven expression method can create various expression input than the other methods. Also, as a primary sensory organ, a hand provides an intuitive experience that enables directly perceiving virtual, holographic objects as if the user tangibly touches them in reality. For instance, Microsoft® HoloLens2 supports a real-time hand tracking so the user wearing the HoloLens2 HMD intuitively plays with the holographic model like grabbing, tossing, or clicking (Microsoft HoloLens, 2023). Likewise, Leap Motion®’s hand tracking controller supports diverse hand/finger gesture-driven interactions along with playful visual effects (Leap Motion, 2016).
Aside from these hand-gesture-driven precedents, research and developments upon the other expression modes such as voice or gazing interaction have been widely carried out in the Human-Computer Interaction (HCI)-related fields. In terms of “Multimodality”, these discrete-like expression modes are combined as a consolidated input module, contributing to expanding the spectrum of interacting with the system and interface (Wollowski et al. 2020). Particularly in the XR realm, integrated with a conventional hand-tracking technology, multimodal input-driven XR experience gradually becomes a technological norm in the industry. Above all, Apple® Vision Pro commercially presents several practical user experiences of navigating the media and information by not only the simplified finger gestures but also the eye-gazing controls and voice-commands (Apple, 2023).
As Apple Vision Pro shows the hybrid interaction methods towards the XR discipline, the usefulness of hybridizing the natural interactions in terms of multimodality has been researched and inevitably become needed for an immersive user experience (Hertel et al. 2021). In the case of adaptively combining the AI agent with the other medium or environments, multimodality concept in inputting sensory could be the key that expands the spectrum of expression methods. For example, Milica Pavlovic et al. suggested the tangible lighting interaction surface for interacting with an AI agent by multimodal inputs such as hand gesture and reactive lighting projections (Pavlovic, 2020). Likewise, compared with the mono-modal interaction method, multimodal input-driven interaction for an AI agent enables to cope with users’ verbal and non-verbal expression methods in a simultaneous way. In particular, by the application of multimodal interaction methods—which accept the user’s natural interactions such as eye-gazing or head-directing motion as a seamless interaction flow—in the XR environment, the quality and heuristic aspects of receiving information via AI agents or interfaces become more interactive and immersive (Rakkolainen et al. 2021). Yet, of course, moderation and optimization on multimodality should be considered in concretizing the multimodal input framework (Margetis et al. 2019). Given all these, appropriate multimodality in accommodating the user’s diverse sensory input has the potential in terms of designing the user experiential aspect of the XR platform into a more user-friendly way.
Fundamentally, this user research aims to investigate the latent usability preference on AI-integrated XR environment. This research intentionally fosters the hand gesture-driven, multimodal communication method in interacting with an AI agent that exists in the virtual home space. In regard to defining the main property of multimodal communication method, it broadly consists of three parts: (1) Hand Gestures; (2) Voice Commands; (3) Eye-Gazing. Based upon these directions, specific gestural motions and verbal expression modes are devised and applied to the user scenario in a form of a mixed communication sequence. That means, in a sense of multimodality, a user in the XR environment interacts with the AI agent and the interface alternately with hand gestures and the other expression methods like voice commanding. Compared to the mono-directional expression experience such as interacting with the interface by solely using hand gestures, this hybrid-fashioned approach has the potential of expanding the spectrum of multi-directional input, and which allows laypersons to get to more easily acclimate to the unfamiliar system by using their preferential expression method. Through iterative user-participatory simulations, this study ultimately explores the possibility of how the AI-agent that either independently exists in the virtual home space or is dependent to the user interface interplays with users by multi-directional expression methods.
2. Method
2.1. Development overview
In regard to the methodological framework for the user simulation and analysis, this study focuses on two major aspects: (1) Development and simulation upon AI agent-driven XR environment based on multimodal sensory input, (2) Participants’ usability preference analysis towards the simulation. To concretize the development process for the user simulation, a developmental workflow prior to the user dataset analysis is sequentially planned (Figure 1). Particularly, establishing the core principles of an AI agent—that either independently exists in the XR environment or dependently exists with the informational interface—is carried out prior to programming the interaction. Stemmed from these established characteristics of an AI agent, its relational facets—such as hand gestural behaviors and its subsequent interface interactions responding to those gestures—are planned and developed accordingly.
Also, in terms of defining a communicative method among the user, the interface and the AI agent, the property of multimodal interaction is established. To intuitively interplay with the AI agent-driven XR environment and interface, as mentioned above, this study mainly concentrates on three aspects as expressive means of natural interactions—Hand Gestures, Voice Commanding, and Eye-Gazing. With these principal criteria, detailed protocols on individual interaction methods are developed and instructed to the simulation participants to interplay with the AI agent and interface.
Based on an established framework that defines the traits of the AI agent and the multimodal interaction on the planning section of Figure 1, a virtual home space and an algorithm for gesture-driven interactions are modeled in Unreal Editor (UE, ver. 5.1.1) as a basic developmental platform. In the Unreal Editor platform, Meta XR plugin (ver. 1.86.0) and its option assets (i.e., hand tracking options provided in UE’s blueprint control panel) are mainly used in programming hand gesture tracking function while wearing the HMD. For the other interaction methods such as voice commanding and eye-gazing, those are not actually developed in this study, and only its interactive response are scripted with a keypad function on UE’s blueprint. That means, by “Wizard of Oz (Nielsen Norman Group, 2022)” method, simulation participants are asked to assume the situation during the session that they are interacting with the voice-control-enabled and eye-gazing-control-enabled AI agent in the XR environment, even though those functions are not assisted in the UE platform and its graphic responses are manually operated by the keypad.
To transmit the model data (virtual home space and interface) to the HMD and receive a user’s hand gesture data from the HMD in real time, the Oculus application (ver. 60.0.0.162.352) installed on the desktop and the HMD serves as a bridge platform between UE and the HMD. For the HMD, this study uses Meta Quest Pro and its controllers are deliberately excluded from the simulation since this study fundamentally pursues the non-controller-based, natural interaction-driven simulation. On the basis of this overall framework, user participatory-driven simulation sessions are conducted, then users’ heuristic datasets regarding the experience over the AI agent-driven XR environment are collected and analyzed in the analysis phase.
2.2. Defining the intelligent agent in the XR environment
In concretizing the characteristics of an AI agent in the XR environment, as mentioned in the overview of Methods section, this study primarily deals with two types of AI agents that either independently or dependently exists in the XR environment. Depending on these established criteria, its corresponding gestural/non-gestural expression methods are devised and its functional subsets are scripted in the blueprint of UE.
As an individual object, this Independent-Type Agent (ITA) is embodied into an orb-like 3D object. Floating in the virtual home space, it is firstly generated at any desired location by opening hand gesture in the XR environment (Figure 2-A). In terms of multimodality at mutual communication, ITA involves engaging interactions through eye-gazing, hand gesture, and voice commands (see Figure 3’s agent classification and its multimodal interaction methods per each sequence). Within the simulation session, as mentioned above, participants are asked to perceive this AI agent as the one that responds to participants’ verbal expressions as voice commands and eye-gazing movement even though its functionality is not actually supported in this simulation. Above this, due to the developmental setting aided by Meta XR plugin within UE, this independent AI agent actually responds to participants’ several hand gestural types. Particularly, mixed with voice commanding usability while interacting with the interface, participants use their hand gestures to control the AI agent’s responsive dialogue like a stop gesture for pausing a dialogue or a swiping gesture for switching a dialogue subject.
This Dependent-Type Agent (DTA) features the dependency on the mutual interaction with a panel-typed interface (Figure 2-B). Same as (1)’s independent AI agent, this agent is rendered into an orb-like 3D object. For the multimodal interaction method, analogous to ITA’s multimodality, DTA is interactive to a participant’s eye-gazing, hand gesture, and voice commanding. Responding to a participant’s hand gestural motions when navigating the 2D panel-based contents on the interface, DTA activated adjacent to the interface panel dynamically adjusts its reference position according to a participant’s shifting eye-gazing direction towards another panel. Also, like the independent AI agent’s preset above, participants are asked to be aware of this as the agent that tracks participants’ eye movement towards a targeting content/thumbnail of a panel interface. In the simulation, participants who wear the Quest Pro are instructed to gaze the other targeting panel of an interface while seeing the content, and the AI agent orb gets regenerated on the top of a panel participants shift their gaze into.
2.3. Designing multimodal interaction method
In specifically defining the property of multimodal sensory input for the interaction with the AI agent and the interface, as broadly mentioned above, this study deals with three sensory inputs—hand gestures, voice commanding, and eye-gazing—as principal behavioral criteria. Based upon those archetypal principles, detailed gestural motions are individually devised. In notionally defining a user’s expressive combination by three established sensory input principles, hand gesture comparatively serves as a primary sensory input element among the other ones since it is the most intuitive, immediate expression method, especially in the case of rapidly flicking the contents or intervening the AI agent’s verbal response. Regarding this consideration, Hirzle et al. explains that the majority of research associated with simulating the AI under the XR preferentially deals with hand gesture-driven interaction than the other methods (Hirzle et al. 2023). Also, utilizing hand and finger as a universal gadget is renowned as an intuitive and natural act of expression—that does not require any intellectual understanding—among the multimodal communication methods, such as pointing at a certain object with a finger (Rakkolainen et al. 2021).
With this hierarchal set-up between sensory inputs, clustering and classifying expressional inputs depending on ITA and DTA was conducted under the user’s representative behavioral patterns (Figure 3). In terms of multimodality, granular properties of each hand gesture, eye-gazing, and voice commanding grouped under a certain behavioral keyword are intermixed altogether as a consolidated communication method in the processes of interacting with the independent, dependent AI agent and the interface.
Fundamentally, this multimodal interaction-driven approach towards the AI agent and interface within the XR environment is related to the latent possibility of technological advance on AI technology. Due to rapid advance of AI capacity, it is expecting that AI-integrated products, platforms and environments will agilely react to a user’s verbal request with a more sophisticated, well-detailed response (Kalla et al. 2023). To satisfy this anticipation, fostering an environment where the real-like interaction with Intelligent Virtual Agent (IVA) can take place is crucial (Guimarães et al. 2020).In particular, for an immersive conversation with IVA, qualitative improvement on the process of communication through effectively tuning the response time is recognized as one of the important points (Wienrich et al. 2018).Likewise, properly fostering the adequate behaviors of the AI agent such as polite verbal and non-verbal manner during the mutual interaction promotes a positive usability result (Zojaji et al. 2020).
The active intervention of the other sensory inputs whilst conversating with the AI agent becomes a significant aspect in terms of effectively moderating the verbal and non-verbal responses the AI agent provides with. In this respect, as Figure 3 shows, we devised four different types of behavioral patterns in which the non-verbal expression methods like hand gestures and eye-gazing are grouped with voice commanding. Particularly, for an effective intervention at the conversational status with the AI agent, stop, swiping, and waving hand gestures are applied to each behavioral pattern, enabling to pause the conversational content the agent is talking about or agilely navigate the other contents. These established hand gestures in the user simulation session get to perform as a gestural moderator that maintains the appropriate interactivity with the AI agent under the XR condition.
3. Experiment Design
3.1. Experiment overview
With the established developmental workflow and multimodal inputs, as mentioned above, a simulation-driven experimentation is concretized grounded in two types of AI agents under the XR condition: (1) AI agent that independently exists in the virtual home space (Independent-Type Agent, ITA), (2) AI agent dependent to a panel-type interface (Dependent-Type Agent, DTA). Based on these principal directions, the overall experiment procedure was specifically designed (Figure 4). Regarding the experiment, it was conducted during October 12th to 19th, 2023, at the UX Research Room in the basement area of Samsung R&D center in Umyeon-dong, Seoul. Experiments were taking approximately one hour on average per participant, and all participants were informed about the overall procedure of the experiment. Lastly, they wrote a consent form regarding the use of user information collected from the experiment. Participant details are explained in the following section.
For the participant detail, a total of 27 participants (Female: 16. Male: 11) participated in the experiment. They consisted of UX designers and VR/AR developers working in Samsung Electronics R&D center, and had no physical, mental issues regarding experiencing the XR environment. After the experiment, they got paid $15(USD) as a participant reward.
To explain about the experiment procedure, a participant is firstly instructed how to interact with the AI agent and the interface in the XR environment by verbal and non-verbal methods. After that, a participant wears the Meta Quest Pro HMD, then goes through the simulations of which the user scenarios are based on two types of AI agents (Figure 5). Also, a participant is guided how to do the gestures or conversate with the AI agent by the promised gestures and dialogues in each sequence, and as mentioned above, a participant is asked to perceive the AI agent as the object that can answer any questions you ask.
In Simulation #1, a participant interacts with the AI agent dependent to a participant’s eye-gazing shift towards the panel interface and voice commanding (Figure 6). By gazing the thumbnail panel, the AI agent gets awaken (Activation), responding to a participant’s eye-gazing shift towards the other content panel (Navigation). In addition, under conversational circumstance with the AI agent, a participant pauses the dialogue the agent is speaking by a stop hand gesture (Pause), switching the conversation topic by a swiping gesture (Navigation), and deactivating the agent by a waving hand gesture (Exit).
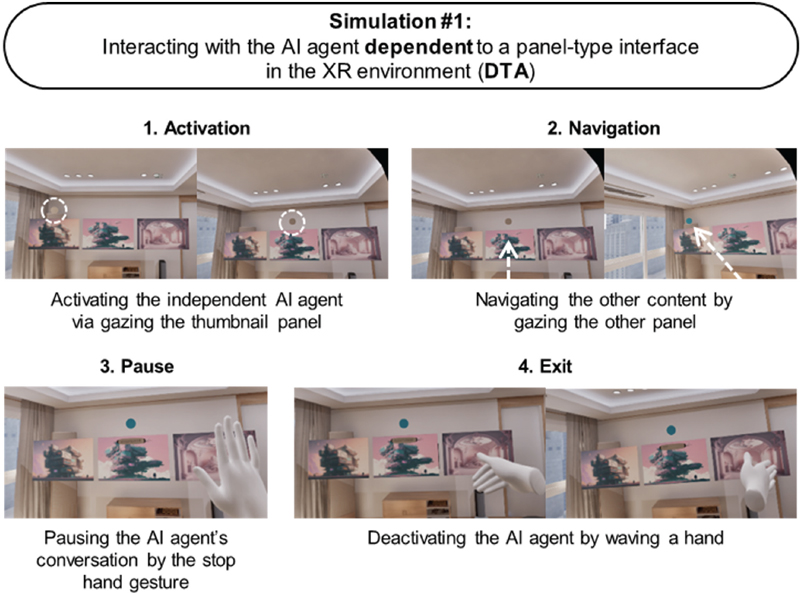
Sequences of interacting with an AI agent dependent to a panel-type interface (conversations between a participant and an AI agent are not visible in these images)
Likewise, in Simulation #2 (Figure 7), a participant activates the independent AI agent by the opening hand gesture (Activation). Following this action, the orb-like AI agent floats in the virtual home space, responding to a participant’s verbal asking. Analogous to Simulation #1’s hand gesture interaction, a participant changes the agent’s conversational response by a swiping hand gesture (Navigation), pausing the dialogue by a stop hand gesture (Pause), and deactivating the agent by a waving hand gesture (Exit).
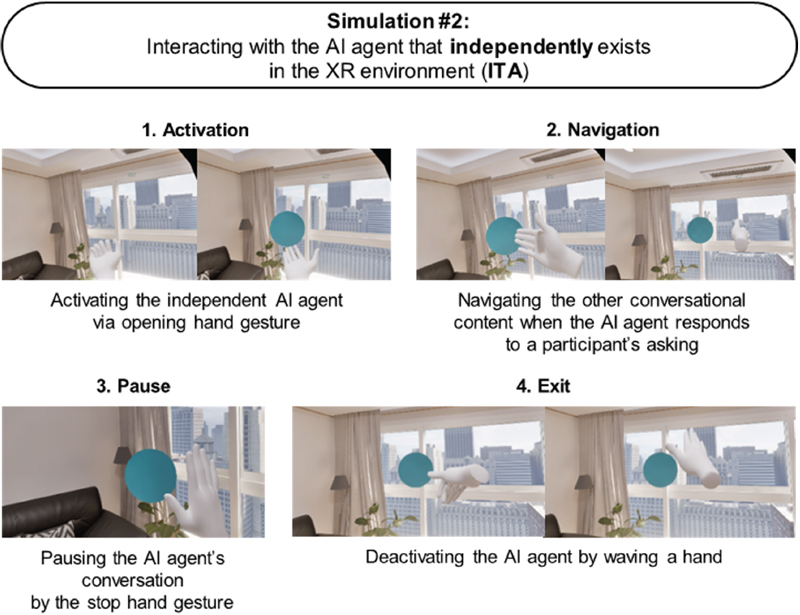
Sequences of interacting with an independent AI agent (conversations between a participant and an AI agent are not visible in these images)
Per each simulation session, a participant is asked to fill out a usability survey regarding the simulation experience (Table 1). After completing all the simulation sessions along with two usability surveys for each simulation, a post-experimental interview is carried out to get the participant’s qualitative feedback on the entire simulations (Table 2). In particular, the analytic tool “User Experience Questionnaire—Short Version (UEQ-S)” was mainly utilized to create a set of scale-based questionnaires in Table 1, in terms of measuring participants’ emotional, experiential feedback towards the simulations. In principle, as a short version of UEQ, this tool measures user experiences via eight criteria of emotional, cognitive expressions (Schrepp et al. 2017). Through this process, user data is analyzed, interpreted into a statistic outcome.

UEQ-S-based scale survey for the usability evaluation per each simulation session. All the terms used in the table are borrowed from Dr. Martin Schrepp’s short UEQ list (Schrepp, 2023)
3.2. User data analysis
In analyzing the collective user data gathered from the simulation sessions and the post-experimental interview, participants’ responses on Table 1’s UEQ-S-based questionnaire are primarily used in this phase for a quantitative analysis. First, based on those UEQ-S user data, TEAM UEQ’s Data Analysis Tool (https://www.ueq-online.org) was utilized to measure the three aspects of participants’ simulation experiences: Pragmatic, Hedonic, and Overall Quality. Also, through this tool’s measurement system, two types of AI agents simulated in the experiment—(1) AI agent that independently exists in the XR environment (ITA), (2) AI agent dependent to a panel-type interface (DTA)—were mutually evaluated based upon TEAM UEQ’s General Benchmark datasheet that covers 468 product evaluations (Schrepp et al. 2017).
Next, participants’ UEQ-S data that reflects two AI agent-driven simulation results is statistically analyzed. For a statistic analysis, ResearchPy (Ver.0.3.5) library was mainly used within Python3.8 environment. Regarding the user data process, participants’ UEQ-S data was gone through the Normality Test, and indicated significant difference between two results on two different AI agent types (Shapiro-Wilk test, all ps< 0.05). Given this interim result, Wilcoxon Signed-Rank Test (WSRT) was conducted to investigate mutual relationship between two AI agent types. In addition, we divided participant responses upon Table 2’s questionnaire into Positive/Negative responses based upon participant reactions and dialogue context (i.e., Per each question, first we directly asked a participant with a question one more time like “Do you feel positive or negative on this?”. After answering to this simple question based upon the main question, a participant answers in detail about the main question of Table 2). With this process, some of participant comments are highlighted in the result section as insightful user experience feedback towards the AI agent-driven multimodal interaction within the XR environment.
4. Result
4.1. Usability feedback analysis on two AI agent types
Regarding participants’ usability responses on Table 1, as mentioned above, participants’ UEQ-S data for two different types of AI agents is analyzed into three aspects: Pragmatic Quality, Hedonic Quality, and Overall Attractiveness. Based upon these three criteria, we measured each agent type’s average value and relative quality rating per criteria (Figure 8). Overall, both ITA and DTA provided with positive user experiences along with “Excellent (mean = 1.81)” and “Good (mean = 1.34)” ratings respectively. Also, the overall attractiveness between two agent types was statistically significant (WSRT, Z = 5.23, p< 0.001). In terms of pragmatic quality, ITA received slightly a more positive response than DTA (Mean_ diff(ITA, DTA) = 0.19), yet no significant difference was found between them (WSRT, Z = 1.27, p = 0.21). In terms of hedonic quality, ITA was positively rated in “Excellent”, surpassing DTA positioned in “Good” (Mean_diff(ITA, DTA) = 0.74). Also, the hedonic quality between two agents showed a significant difference (WSRT, Z = 5.95, p< 0.001). To summarize, participants in the simulation mostly showed positive responses on both ITA and DTA types, and commonly responded that ITA is relatively more enjoyable and attractive to interact with than DTA.
Next, regarding the user preference on agent types, we investigated participant feedback on Table 2’s question #4 (Figure 9). 51.9% of participants responded positive towards harnessing both agent types, and a common opinion among them was that both agent types would be necessarily needed since those agents could be both used in different types of situational contexts. Particularly, they mostly wanted to individually make use of each AI agent depending on each specific task (“[P02] I think that a dependent-type agent would be good for office tasks, and I would utilize an independent-type agent for when I would like to comfortably watch videos.”; “[P11] I would like to use both agents. A dependent-type agent would be convenient as I am in the situation of navigating the media on the interface, yet simultaneously I would like to use an independent-type agent as well since the experience of interacting with the independent-type agent in the virtual space as a virtual butler seems fun.”). Participants who chose only ITA (37.0%) mostly commented that DTA’s activating motion responsive to a participant’s eye-gazing is burdensome and disruptive (“[P13] An agent responding to my eye-gazing visually distracted my attention, because it unnecessarily showed up even though I felt that the agent was not needed when navigating the contents.”). Lastly, 11.1% of entire participants responded positive to only using DTA, since it is a relatively faster way to directly achieve the result on what they would like to get by the aid of an AI agent, when navigating the contents on the interface (“[P19] I like using the dependent-type because it quickly provides with answers based on the targeting content I stare at and inquire about.”).
4.2. Comparison with the mono-modal AI agent, and the analysis on the intension of reuse
In comparison with the other AI agent types in the mobile device platform such as smartphones and smart tablets, 92.6% of participants responded positive on AI agent experiences (ITA, DTA) in simulations (Figure 10-1). Regarding the reason, the most frequently mentioned comment was that participants were able to clearly sense the agent as a tangible object, compared to the one embedded in the mobile device and which is relatively hard to sense its presence (“[P07] Unlike the interaction method with the AI in the mobile smart device, the AI agent that exists in the space makes me feel a more enhanced sense of interaction.”; “[P23] The previous AI agent in the mobile device was more like an indicator, making it more difficult to perceive its presence. With this AI agent, I felt like it exists right next to me.”).
Secondly, participants responded positive on the usability of interacting with the AI agent by either eye-gazing or a simple hand gesture than manually doing a wake-up word on it (“[P14] The AI agent embedded in the mobile device makes me feel constraint to the device location since it cannot be relocated according to my call. But, regarding the AI agents I experienced in the simulations, what was good for me was that I can easily call the agents to wherever I want, with no disturbance on the screen panel I am watching on the interface.”). Whereas, 7.4% of participants responded negative on the AI agent types conducted in the simulations, rather expressing a convenience on voice-driven, conventional AI agent embedded in the mobile device (“[P09] I do not feel necessarily needed of those AI agents that exist in the virtual home space. I feel it is redundant to the usability of the currently existing AI agent in the mobile device in terms of adding just a few more inputs to the conventional voice commanding method.”; “[P25] I could not feel any difference to the current AI agent that exists in the mobile device since the most of crucial commands were somehow carried out by voice commanding in the simulation.”).
The results of aforementioned participant responses are linked with the willingness of reusing the proposed AI agents in a same ratio (Figure 10-2). The participants who responded positive on reusing the proposed AI agents (92.6%) commented that interacting with the virtual environment via AI agents was fascinating and relatively easier than controlling via HMD’s hand controllers. On the other hand, the participants who responded negative (7.4%) commented that the proposed AI agent in simulation is analogous to the one embedded in the mobile device in terms of interacting with the agent via voice commanding. They rather said that the current voice-commanding-driven AI agent is relatively more intuitive and convenient than the proposed one. These findings imply that the multimodal input-driven AI agent present in the virtual home space relatively fostered the user-friendly, seamless interaction with the participants, compared to the conventional, mono-modal input-driven AI agent.
4.3. User convenience on the multimodal input-driven interaction method
Regarding the Table2’s question #3, 74.1% of participants responded positive on the interaction method with the AI agents (Figure 11). Participants commented that the multimodal input-driven interaction method used in the simulation is intuitive and largely convenient especially in interplaying with the AI agent in the XR environment (“[P01] It was quite convenient to interact with the AI agent by not only a hand gesture but also an eye-gazing experience, which I have not been able to do in the mobile device environment.”). In addition, some participants added a mention that this multimodal input-driven method is what they previously experienced in the research field as a prototyped technology so it was familiar to experience in the simulation (“[P22] The multimodal input-driven methods such as pointing the object by eye-gazing as well as one-handed gestures was familiar for me to experience.”). On the other hand, 25.9% of participants responded negative on the multimodal input-driven interaction method. The participants who responded negative commented that the hand swiping gesture that consists of left and right hand swiping motion seems confusing with the waving hand gesture that signifies deactivating the AI agents (“[P12] A swiping hand gesture for navigating contents is confusing with a waving hand gesture even though I understood how those two different gestures work. Because of this, I think I was waving my hand cluelessly when trying to turn off the agent.). Also, two of those participants who responded negative commented that gesture-based interaction is uncomfortable, and rather advocated the use of voice commanding-driven method (“[P19] I did not feel absolutely needed for using a hand gesture method when it comes to interacting with the AI agent although it was not that uncomfortable to use for the interaction. I rather prefer to do a voice commanding to my AI agent because it is easier to do so.”).
For the question #5 of Table 2, the most of participants mentioned about the improvement points that should be considered for a next version of this study’s AI agent simulation. In particular, they commented about improving the interface’s interactivity regarding interplaying with the AI agent whilst navigating the contents on the interface (“[P03] The interface should be improved into a way that facilitates to see what kinds of commanding features are available as a form of an assistive interface especially on the situation of gazing the targeting panel.”). Additionally, they commented the latent needs of more tangibly interacting with the AI agents (“[P06] I think it would be fascinating if the agent responds to my haptic touch with more tangible reactions like morphing the shape towards my physical hand gestures like grabbing or pushing.”).
5. Conclusion
This study explores how two different types of AI agents in the XR environment interact with the user by the multimodal input-driven interaction methods. Through the participatory simulations, this study experimentally investigated the possibility that the AI agents proposed in this project are adaptively interactive with participants. In addition, through quantitative and qualitative analysis of surveys, we clarified participants’ inherent preferences regarding the usability of two types of AI agents in the XR environments. The analytical results provided clues on how multimodal input-driven interactivity should be practically developed in a user-friendly manner.
In particular, regarding the analysis results, this study apparently shows that most of participants prefer to experience the AI agent perceived as an actually existing one despite of its virtual presence in the XR environment. In interplaying with the agents, participants pursued not only the practical usefulness but also the playfulness in terms of enjoying the interaction itself with the agents by using diverse hand gestures and so on. Secondly, regarding the simulations on two AI agent types—ITA and DTA, participants mostly prefer to alternatively make use of both agent types in the XR environment since ITA can be activated anywhere participants want and DTA conveniently responds to participants’ content navigation within the panel interface. Thirdly, the majority of participants were actually familiar with experiencing the multimodal input-driven interaction method that includes hand gestures, eye-gazing, and voice-commanding. Simultaneously, an elaborate development on multimodal input system for interacting with the AI agent in the XR environment is also demanded for a next revision.
In prototyping the AI agents’ multimodality based on establishing the properties of natural interactions and development, this study has several technical, methodological limitations. Firstly, as mentioned in the method section, in the simulation participants were asked to perceive the AI agent as the one that assists voice recognition and eye-tracking even though it only tracks participants’ hand gestural motions. In terms of Wizard of Oz method, except the hand gestural expressions, participants were asked to conversate with the AI agents based on the dialogue script and also were asked to gaze the promised panel of the interface when activating DTA responsive to a participant’s eye-gazing. For these promised inputs, AI agents’ outputs such as conversational response and activation were programmed and manually carried out by programmed keypads in Unreal Editor. Therefore, in order to overcome this technical limitation, the attempts of developing a real, workable prototype are highly demanded for a next phase of a research. It would take tremendously lots of time and human resources to embody the AI agent that enables to actually recognize the voice and responds to a user’s eye-gazing, yet, through this kind of workable prototype, it is expectable that the true empirical research on AI agents that exist in the XR environment would become feasible.
In addition, in terms of optimizing the gestural inputs when interacting with the AI agents, mitigating the similarity between hand gestures should be dealt with in the next research. As the participant [P12] commented in 5.3. Section, similar gestural inputs aiming for different interactions could confuse users’ perception and consequently lead to the overall deterioration of usability. Through iterative user feedback and testing sessions on gestural inputs, this similarity issue needs to be fixed and optimized for a clarified interaction.
Lastly, in collecting participant feedback, this study relied upon participants’ experiential aspect when comparing the proposed AI agent with the currently existing AI agent embedded in the mobile devices. For an accurate, practical comparison, comparative studies based upon the investigation on multimodal input-driven and mono-modal input-driven interaction methods should be considered in the next research. This comparative investigation would potentially contribute to the other adjacent HCI research regarding multimodality-driven AI interactions.
Acknowledgments
This work was done by Samsung Electronics.
Notes
Copyright : This is an Open Access article distributed under the terms of the Creative Commons Attribution Non-Commercial License (http://creativecommons.org/licenses/by-nc/3.0/), which permits unrestricted educational and non-commercial use, provided the original work is properly cited.
References
- Apple. (2023, Jun 6). Introducing Apple Vision Pro [Video]. YouTube. Retrieved from: https://www.youtube.com/watch?v=TX9qSaGXFyg.
- Apple. (2023). Apple Vision Pro [Website]. Retrieved from: https://www.apple.com/apple-vision-pro/.
-
Chu, M., & Begole, B. (2010). Natural and implicit information-seeking cues in responsive technology. In Human-Centric Interfaces for Ambient Intelligence (pp. 415-452). Academic Press..
[https://doi.org/10.1016/B978-0-12-374708-2.00017-6]
-
Guimarães, M., Prada, R., Santos, P. A., Dias, J., Jhala, A., & Mascarenhas, S. (2020, October). The Impact of Virtual Reality in the Social Presence of a Virtual Agent. In Proceedings of the 20th ACM International Conference on Intelligent Virtual Agents (pp. 1-8)..
[https://doi.org/10.1145/3383652.3423879]
-
Hertel, J., Karaosmanoglu, S., Schmidt, S., Bräker, J., Semmann, M., & Steinicke, F. (2021, October). A taxonomy of interaction techniques for immersive augmented reality based on an iterative literature review. In 2021 IEEE international symposium on mixed and augmented reality (ISMAR) (pp. 431-440). IEEE..
[https://doi.org/10.1109/ISMAR52148.2021.00060]
-
Hirzle, T., Müller, F., Draxler, F., Schmitz, M., Knierim, P., & Hornbæk, K. (2023, April). When XR and AI Meet - A Scoping Review on Extended Reality and Artificial Intelligence. In Proceedings of the 2023 CHI Conference on Human Factors in Computing Systems (pp. 1-45)..
[https://doi.org/10.1145/3544548.3581072]
- Kalla, D., Smith, N., Samaah, F., & Kuraku, S. (2023). Study and Analysis of Chat GPT and its Impact on Different Fields of Study. International Journal of Innovative Science and Research Technology, 8(3). Available at: https://ssrn.com/abstract=4402499.
- Leap Motion. (2016, Feb 17). Leap Motion: Orion [Video]. YouTube. Retrieved from: https://www.youtube.com/watch?v=rnlCGw-0R8g.
-
Margetis, G., Papagiannakis, G., & Stephanidis, C. (2019). Realistic natural interaction with virtual statues in x-reality environments. The International Archives of the Photogrammetry, Remote Sensing and Spatial Information Sciences, 42, (pp. 801-808)..
[https://doi.org/10.5194/isprs-archives-XLII-2-W11-801-2019]
- Meta Quest. (2019, Nov 5). Defy Reality [Video]. YouTube. Retrieved from: https://www.youtube.com/watch?v=Pxht1IJAJr0..
- Microsoft HoloLens. (2023, Sep 28). Enhance frontline worker experience anytime, anywhere with Microsoft HoloLens 2 & Mixed Reality Apps [Video]. YouTube. Retrieved from: https://www.youtube.com/watch?v=pIsjVaqdNpc.
- Nielsen Norman Group. (2022). The Wizard of Oz Method in UX [Website]. Retrieved from: https://www.nngroup.com/articles/wizard-of-oz/.
-
Pavlovic, M., Colombo, S., Lim, Y., & Casalegno, F. (2020). Exploring Gesture-Based Tangible Interactions with a Lighting AI Agent. In Human Interaction and Emerging Technologies: Proceedings of the 1st International Conference on Human Interaction and Emerging Technologies (IHIET 2019), August 22-24, 2019, Nice, France (pp. 434-440). Springer International Publishing..
[https://doi.org/10.1007/978-3-030-25629-6_67]
-
Rakkolainen, I., Farooq, A., Kangas, J., Hakulinen, J., Rantala, J., Turunen, M., & Raisamo, R. (2021). Technologies for Multimodal Interaction in Extended Reality-A Scoping Review. Multimodal Technologies and Interaction, 5(12), 81..
[https://doi.org/10.3390/mti5120081]
-
Schrepp, M., Hinderks, A., & Thomaschewski, J. (2017). Design and Evaluation of a Short Version of the User Experience Questionnaire (UEQ-S). International Journal of Interactive Multimedia and Artificial Intelligence. 4(6), (pp. 103-108)..
[https://doi.org/10.9781/ijimai.2017.09.001]
- Schrepp, M. (2023). User Experience Questionnaire Handbook (Version 11 - 12.09.2023) [PDF]. Retrieved from: https://www.ueq-online.org/Material/Handbook.pdf.
- Team UEQ. (2018). UEQ User Experience Questionnaire [Website]. Retrieved from: https://www.ueq-online.org/.
-
Wienrich, C., Gross, R., Kretschmer, F., & Müller-Plath, G. (2018). Developing and Proving a Framework for Reaction Time Experiments in VR to Objectively Measure Social Interaction with Virtual Agents. In 2018 IEEE Conference on Virtual Reality and 3D User Interfaces (VR) (pp. 191-198). IEEE..
[https://doi.org/10.1109/VR.2018.8446352]
-
Wienrich, C., & Latoschik, M. E. (2021). extended artificial intelligence: New prospects of human-ai interaction research. Frontiers in Virtual Reality, 2, 686783..
[https://doi.org/10.3389/frvir.2021.686783]
-
Wollowski, M., Bath, T., Brusniak, S., Crowell, M., Dong, S., Knierman, J., Panfil, W., Park, S., Schmidt, M., & Suvarna, A. (2020). Chapter 20 - Constructing mutual context in human-robot collaborative problem solving with multimodal input. In Human-Machine Shared Contexts (pp. 399-420). Academic Press..
[https://doi.org/10.1016/B978-0-12-820543-3.00020-1]
-
Zojaji, S., Peters, C., & Pelachaud. C. (2020, October). Influence of virtual agent politeness behaviors on how users join small conversational groups. In Proceedings of the 20th ACM International Conference on Intelligent Virtual Agents (pp. 1-8)..
[https://doi.org/10.1145/3383652.3423917]