
Investigating the Emotional Responses to Generative Art
Abstract
Background Generative art, which includes the development of artworks using algorithms and emergent behaviour, is receiving recognition for its tendency to evoke emotion. A vast spectrum of emotional responses may be elicited by generative art by applying dynamic patterns, compositional decisions, and movement. However, a lack of understanding currently exists regarding the emotional impact of creative expression.
Methods In the first stage, data collection followed a research methodology that utilised a campus-based survey conducted with eighteen undergraduate design students who were reached through different university recruitment methods. The survey used a mix of quantitative and qualitative data collection methods since it involved viewing generative art stimuli, choosing relevant emotional descriptors and providing novel understandings of participants’ emotions through open-ended response approaches. The analysis adopted a descriptive statistical approach to determine the patterns and trends of the reported emotional responses. In the second stage, a group of six participants assessed three generated artworks, utilising techniques such as word cloud analysis and eye-tracking technology. The visual attention patterns and audio responses were investigated to gain insight into emotional experiences utilising eye-tracking technology. Participants employed word clouds to represent their emotional state.
Results The analysis of eye-tracking data and verbal responses established that participants experienced different emotional reactions to the artworks. Different emotions — including engagement, optimism, analytical stance, neutrality, mixed emotions, and introspection — were experienced during phases of participants’ interactions with the system. The generated artworks prompted emotions of interest and excitement through their dynamic transitions and visual attractiveness. The resizing of graphical components and manipulations and movement of grid units prompted neutral emotions in certain cases.
Conclusions The researchers also noticed that the patterns output by the algorithms, colour combinations, and symbolic representations had the potential to induce specific emotional states. This research could also lead to the development of more sophisticated communication applications and improved audience engagement. Future research and collaboration between scientists and artists are needed to fully understand the neural underpinnings of emotional reactions to generative art.
Keywords:
Generative Art, Emotional Response, Algorithmic Patterns, Design Elements, Eye-tracking1. Introduction
Generative art is an interdisciplinary subject in which algorithms are used to produce visual or audio artworks; they often involve randomness and growth-like patterns generated by the algorithms. It is an interdisciplinary field which gathers art, design, mathematics, and computer science to create unique and constantly changing experiences. The unexpected emergent behaviours, spontaneous growth, or dynamic patterns in generative art are full of emotions. The interaction among the computational processes, choices in composition, colour palettes and movement can elicit emotions of interest, wonder, serenity or reflection. The research on how generative artworks can influence the emotional states of audiences provides new perspectives on the impact of artworks on the emotions of audiences and allows creators to purposefully elicit specific emotions (e.g., passive or active). The understanding of the emotional effects of generative art by artists and designers – both from the point of view of the emotions that these evoke and the understanding of the emotional states they evoke – can enable the creators to make more meaningful and engaging works of art. A large research gap exists on how generative art impacts audiences’ emotions, even as this art form garners more and more attention. Although a few studies exist on the emotional effects of static artworks and traditional artistic formats, the specific mechanisms and design elements through which generative art might elicit emotions have not been studied. By means of algorithmic patterns, colour combinations, symbolic representations, and other sometimes subtle and sometimes extreme design elements that somehow capture or express emotions, generative art is unique and warrants further research to help fill a gap in understanding. There is a paucity of research where eye-tracking data and any vocal feedback from audiences were used to gain insight into audiences’ perceiving and emotional responses to generative artworks.
2. Literature Review
2. 1. Prior Works on Generative Art, Emotion, and Their Intersections
Research on the intersection between generative art and emotions has interesting results. AI-created art can elicit and represent human emotions. (Alvarez-Melis, 2017; Lee et al., 2023). Jha et al., (2021) take it to another level and compare the emotion of machine-generated art to human emotions and historical ideas on art. Fundamental articles on the role of emotions in art (Ristić & Milošević, 2022). Matravers (1998) explores the underlining of the role of emotional representation. The association of emotions with art, as a form of specialisation, is addressed by emphasising valence, arousal, and familiarity having predictive power in the context of creativity. Sullivan and Herzfeld-Schild (2018) take a broader view by investigating the cultural and historical relevance of artistic expressions of emotion. Researchers have shown that generative art has a remarkable ability to make people feel things.
AI-generated art is able to represent human emotions and trigger self-expression (Lee, 2023). Emotional experience, high and low valence and arousal, predict creativity in generative processes (Ristić 2022). Emotions can be transformed into art with a class-conditioned generative adversarial network (Alvarez-Melis 2017). Artistic emotions, among which beauty is prominent, are fundamental for emotional expression and transfer (Thagard 2019). Emotion can be represented in art with a narrative form or an abstract structure (Barringer, 2016). Emotions are a form of artistic medium that defines art (Suneson, 2018).
2. 2. Theories on Emotional Responses to Visual Stimuli in Art
Numerous significant findings have resulted from extensive research into the study of emotional responses to visual stimuli in art. Visual art stimuli mostly elicited positive emotional reactions. According to Cheung et al.,2019) research alluring commercial stimuli alone caused positive responses. In particular, when it comes to abstract art, Else et al.(2015) offered more evidence that creative competency may impact emotional responses. The importance of low-level visual qualities in generating persistent emotional responses was highlighted (Van Paasschen et al. 2014), while Finisguerra et al., (2021) emphasised the same for motor modelling. To understand how people react to audiences depicted in artworks, Kesner and Horáček (2017) developed a thorough framework that focuses on the impact of contextual factors and personal characteristics. Miu et al., (2016) compared emotional reactions to art and music. The results revealed variations in the frequency and fundamental causes of aesthetic perceptions. In addition, in 2022, Lee expanded upon Juslin and Västfjäll’s (2008) findings into the processes that elicit emotions in music, applying them to the domain of visual stimuli. Lee discovered six distinct methodologies for eliciting emotional responses to visual aesthetic stimuli.
3. Research Objectives
This study aims to explore how generative art evokes emotions in audiences. Notably, an unexplored gap in understanding the emotional effect of generative art is intended to address and investigate the components within generative art that elicit emotional reactions.
4. Conceptual Framework that Supports Generative Art and the Emotional Response
Various disciplines, including psychology, neuroscience, aesthetics, and computer science, influence generative art and emotional response. Theories of aesthetics like expressiveness and the formalist approach emphasise the idea that forms, colours, and compositions intrinsically evoke feelings. Many theories provide light on the nature and experience of emotions; examples include cognitive appraisal theory and the fundamental emotion theory. The psychophysiological approach examines physiological indicators of emotional reactions, including skin conductance, facial expressions, and heart rate, to comprehend the fundamental processes governing emotional experiences and their correlation with generative art. According to theories of embodied cognition, the cognitive processes, emotional reactions, and physiological sensations are interdependent. The interplay among motor actions, emotional states, and sensory perceptions influences personal engagement with and reaction to generative art, underscoring the corporeal character of emotional encounters. It is assumed that practitioners and researchers can investigate the cognitive processes and neurophysiological correlates of emotional responses, and how generative art might influence emotions by combining these theoretical concepts. With this knowledge, generative art could be designed to prompt specific emotional states while providing enchanting and moving experiences for audiences.
There are a variety of elements in generative art that may prompt emotional reactions in audiences. These elements may include, but are not limited to the following (shown in Figure 1):
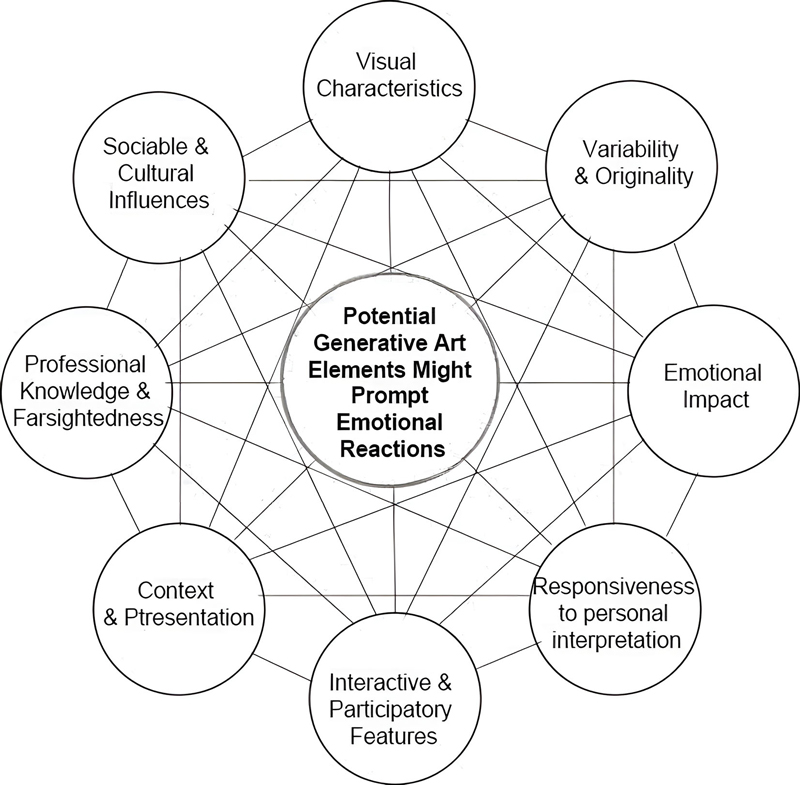
Figure that graphed the potential relationships between elements of generative art that might provoke discernible emotional reactions
- 1. Visual characteristics
- 2. Variability and originality
- 3. Emotional impact
- 4. Responsiveness to personal interpretation (Rank, 1978; Rotenberg,1985).
- 5. Interactive and participatory features (Lukichev et al., 2019).
- 6. Context and presentation
- 7. Professional knowledge and farsightedness (Boden & Edmonds, 2019).
- 8. Sociable and cultural influences (Spaid, 2020).
5 Methodology
5. 1. Usage of Survey and Eye-motion Tracking Tools
The objective of the present study was to explore the emotional states of undergraduate students after viewing the three different generative art stimuli labelled as No.1, No. 2, and No. 3. The survey was implemented on campus, utilising eighteen participants recruited from undergraduate students who were at different years of their design studies. The participants were recruited through university channels that included email announcements, social media postings, and flyers posted on campus. All persons who agreed to participate were presented with written informed consent concerning the purpose of the survey, its procedures, and participants’ rights. This measure established transparency and intellectual integrity. The survey obtained both quantitative and qualitative findings. First, participants provided their characteristics respondent, such as the year of their study. Then, participants viewed three generative art stimuli on the computer screen randomly and had the opportunity to free-view each artwork. After viewing, participants were asked to choose one of the offered descriptions that they believed most appropriately captured what they felt after seeing the image. The emotional descriptors varied among high-energy positive emotions, neutral calm states, and negative emotions. To enrich the understanding of respondents’ perceptions, the survey contained an open-ended question that enabled participants to write down anything they wanted about the artwork. Analysis of data was conducted using descriptive statistics, which aimed to identify the patterns and trends in the emotional responses reported by the participants. It was important to establish the pattern and realise the most frequent descriptors. This survey strictly adhered to the ethical rigour requirements for the study involving human participants. It is also important to mention limitations such as a small sample potentially affecting generalisability; obtaining data differently to exclude respondents’ bias would be beneficial. This survey established an initial stage in identifying the emotional states that undergraduate students experience when viewing these kinds of generative art stimuli. The outcomes can also facilitate the work of artists, curators, and educators.
It is important to reveal that the emotional reactions provoked by individual components of generative art are heavily dependent on individual differences, personal proclivities and subjective interpretations, such that audiences of generative art might find themselves feeling pretty much anything, as these coalesce and interact. Eye-motion tracking is a research tool used to measure participants’ attention and visual focus while they are shown different generative art stimulation. It works by recording and analysing the movement of a participant’s eye or eyes, and data can be gathered showing the parts of the art that received the greatest visual attention. in addition, there are different research methods for capturing participants’ verbal feedback on their emotional responses, such as a word cloud, in which participants provide written or spoken descriptions of their emotional experiences to generative art stimuli. Word clouds are created after participants’ responses are processed to form a visual representation using different-sized words to indicate their frequency of occurrence. This technique provides insight into the common emotional themes or descriptive terms used by participants and offers qualitative insights into the emotional impact of generative art. By combining these research methods, the study attempts to provide both quantitative and qualitative data on participants’ emotional responses. Rather than relying solely on subjective measures of emotional states (i.e., participants self-reporting their feelings), eye-motion tracking ensures that measurements of their visual focus are objective. Similarly, the use of word clouds provides a non-verbal medium for participants to express their emotional states; and, the combination of a word cloud and eye-motion tracking facilitates a more comprehensive understanding of how generative art elicits the emotions it does. Three generative art stimuli created by Prof Kyuha Shim [Q] were presented to the participants as introduced in the following table.
5. 2. Participants
A sample of participants (n=6) were recruited and asked to rate their emotional response using the eye-movement scale. Participants were provided with a graphical representation of emotions and were shown how eye movement represents various levels of arousal and valence. They were asked to select or indicate the appropriate point on the scale that best corresponded to their emotional experience based on the task/stimulus presented. Ratings were collected for the grid initially and visual parameters were recorded and inherent in two numerical values, representing the emotional state of each participant.
6. Result
The obtained data from the participant survey also reveals the emotional responses to various generative art stimuli, namely, No. 1, No. 2, and No. 3. With regard to generative art stimuli No. 1, most of the majority descriptions revealed it was preferred by the Year 3 students, which shows that more experienced undergraduates are more engaged and interested in the given a generative art stimulus. Among the emotional responses, the high-energy, pleasant option was the choice of most participants, which indicates that the work successfully captured their attention and imagination, creating an impression of dynamic energy. In addition to this, the great variety of labels for this generative art stimulus, such as ‘lively,’ ‘illusory,’ ‘colourful,’ ‘sweet,’ and ‘magical,’ also highlights the multifaceted and engaging nature of generative art stimuli No. 1. Contrary to it, the reactions to Generative art stimuli No. 2 were more uniformly distributed among different emotional categories, with a minimal trend to neutral, calm. On the whole, participant descriptions of this work seem to evoke a sense of serene, introversion and technical nature ‘sad,’ ‘quiet,’ ‘peaceful,’ and ‘tech.’ This indicates a more retrospective and less bright response evoked by Generative art stimuli No. 2. Finally, the reaction to Generative art stimuli No. 3 demonstrates a preponderance of high–energy, pleasant reactions and a balance of neutral high-energy reactions. ‘happy,’ ‘cheerful,’ ‘dynamic,’ ‘colourful,’ and ‘comfortable’ labels show that this generative art stimuli disposes the participants to vibrancy, energy, and joy similar to generative art stimuli No. 1. Overall, one can see that each generative art stimuli evoked diverse emotional responses among the audience – joyful and pleasant, calm and introverted, and a combination of both. Therefore, the artists, and in particular the curators, should take this finding into account to flexibly represent their works for maximum audience engagement based on the drilling view.
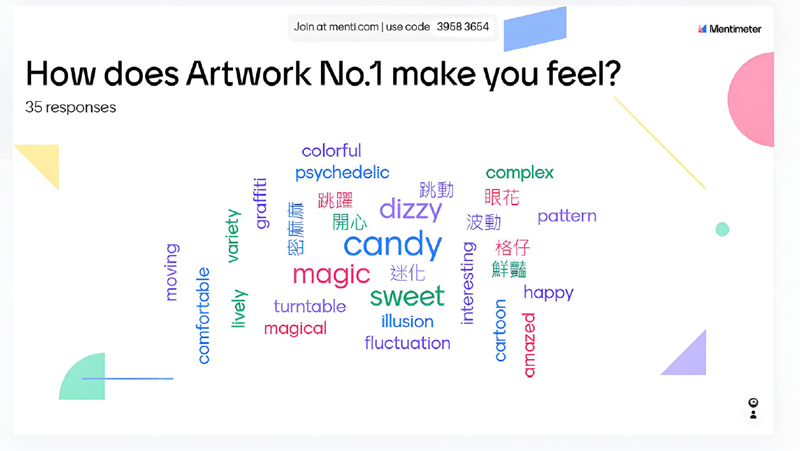
A sample presentation slide guided the participants to select types of emotion while viewing the three generative art stimuli
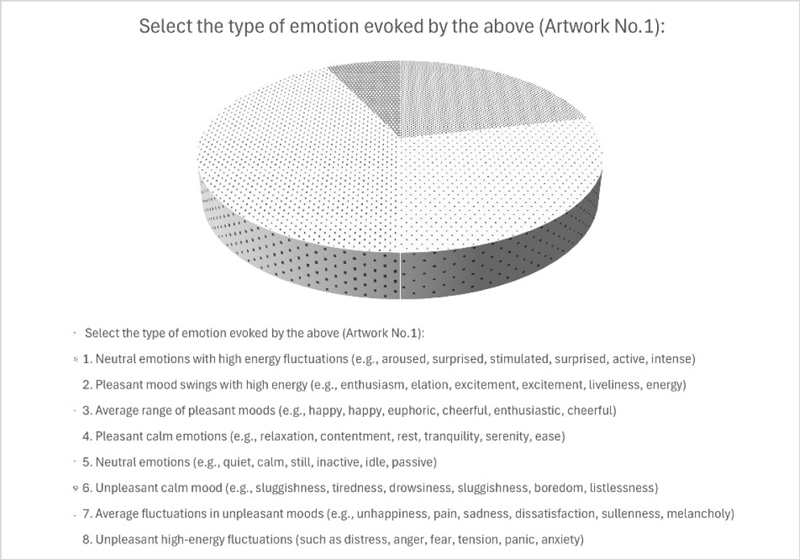
Sample chart that shows the selected types of emotion by participants while they were viewing the three generative art stimuli
Eye-tracking data was collected from Participants P1-P6 during the study to examine their gaze patterns and visual attention (Figure 8-10). By utilising an eye-tracking tool, the researchers were able to record and analyse the participants’ eye movements about the dynamic transitions, scaling of graphic elements, and computational morphing of visual parameters in the three selected generative artworks. Participant ID P1 exhibited a gaze focused on the centre of the grid initially (400, 300), later shifting to the changing number of grid cells (410, 305). Their attention was then directed towards the dynamic transitions of graphic elements (415, 310) and subsequently focused on the computational morphing of visual parameters (420, 305). Participant ID P2 demonstrated a gaze directed towards the changing number of grid cells (380, 280) before transitioning to the scaling of graphic elements (385, 275). They further examined the dynamic transitions of form and colour (390, 280) and observed the computational morphing of visual parameters (395, 285). Participant ID P3 gazed at the dynamic transitions of graphic elements (430, 310) and then at the changing number of grid cells (420, 305) before directing their attention to the computational morphing of visual parameters (415, 310). Finally, they gazed at the scaling of graphic elements (410, 315). Participant ID P4 exhibited a gaze focused on the dynamic transitions of form and colour (400, 320), transitioning towards the changing number of grid cells (405, 315). They also observed the scaling of graphic elements (410, 320) and analysed the computational morphing of visual parameters (415, 325). Participant ID P5 demonstrated a gaze directed towards the scaling of graphic elements (420, 315) and transitioned towards the changing number of grid cells (415, 310). They then analysed the computational morphing of visual parameters (410, 305) and subsequently focused on the dynamic transitions of form and colour (405, 300). Participant ID P6 exhibited a gaze focused on the changing number of grid cells (390, 300) before shifting their attention towards the scaling of graphic elements (385, 295). They then observed the computational morphing of visual parameters (380, 290) and analysed the dynamic transitions of form and colour (375, 285). The eye-tracking data provides insights into the participants’ visual attention and gaze patterns while observing the three selected generative artworks. It offers researchers an objective means to study the participants’ focus areas and reactions to the specific visual stimuli and transitions employed in the artwork. This analysis of eye-tracking data contributes to the academic understanding of how individuals engage with and respond to visual stimuli in generative art compositions. The summary results from participants of Table 2 are represented above in relation to their emotional responses towards the three generative art stimuli. The data shows a clear trend of high-energy, pleasant responses to generative art stimuli No. 1 and No. 3, with average emotional intensities of 4.23 and 4.20 respectively. In contrast, generative art stimuli No. 2 elicited predominantly neutral or low-energy responses, with an average emotional intensity of 2.70. These results support our earlier observations and provide a more granular view of individual participant reactions.
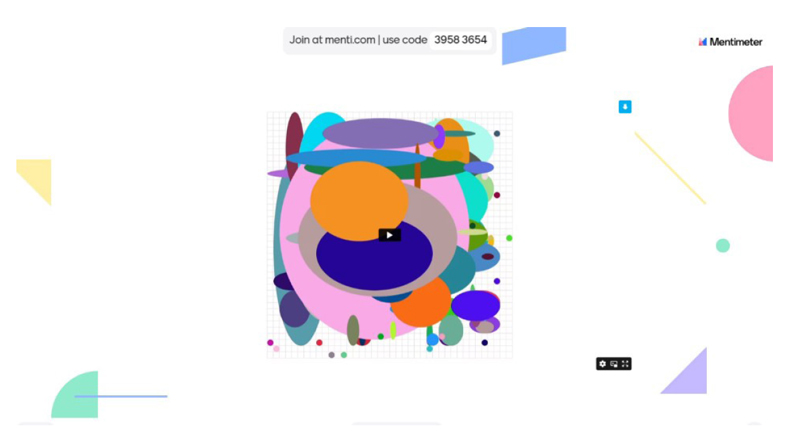
Sample presentation slide guided the participants to conduct eye-movement tracking on three generative art stimuli
The heatmap analysis of the generative art stimuli No. 1 allowed the identification of multiple key features indicating the visual engagement of the participants. First, the heatmap shows that the central focus of the object, identified at coordinates (400, 300) was the location of the highest concentration of visual attention –defined by the hot red area. In this way, it can be said that the very centre of the composition was the most engaging for the viewers. Second, it can be seen that the areas of shifting along their axes, in place, a change in the number of grid cells are noted, registered at coordinates (410, 305), are characterised by a moderate-to-high level of viewers’ attention, indicated by the yellow to red colours. Therefore, we can conclude that the participants’ gaze is mainly focused on the changing grid structure of the object. Lastly, we can see that the regions of constant motion or transition of shape or colour, such as that identified at the coordinates (415, 310), are forming certain yellow patches, demonstrating stable viewers’ attention. On the whole, it can be seen that the majority of the peripheral areas of the visual object are presented in blue or green, identifying the lowest attention rate; at the same time, these areas were homogenous and without movement. In this way, the heat map data supports the conclusion that the viewers focused on the central composition and moving elements of the generative art stimuli No. 1, which is consistent with the high-energy and pleasant emotional responses registered in the survey.
The results suggest that a generative approach where computational morphing, scaling methods, symbolic meaning, colour combinations, and personalisation are incorporated could effectively manipulate a set of emotions. This could generate visually arousing experiences that captivate the interests of people and prompt favourable affective responses. The incremental transformation or manipulation of visual parameters over time could amplify the affective power of generative art with the aid of computational morphing techniques. This could potentially lead to a stronger affective bonding with the artwork since it can invoke curiosity and pull people into a broader range of conflicting emotions. Decisions on altering visual objects’ dimensions, sizes and zooming can also mediate an affective experience. Thus, scaling methods could illicit a more deliberate affective involvement on the part of the audiences. For generative art, the meaning of signs and colours is simply rhetoric. Cultural or narrative implications can be referenced through symbolic significance, a combination of deep concepts with masterfully executed artworks for the implications. In addition, colour itself has both cultural meanings and emotional arousal. It can make a wrong impression for a picture to elicit emotions that its original character never intended. To understand the emotional impact of art, one has to consider those predispositions of mood and background that audiences bring from their pasts. Given the direction that is useful for people taken in but concerned for the future, appreciating the effects of generative artworks, bottom-up experiments, and iterative design is vital. What was keeping the fact that people felt robbed of their emotions away from this era in art? One way to get more control over emotional experiences is by continuously testing and refining based on participant feedback. As a final positive note, generative art may make people feel something, and it can also show artists how to produce superlative experiences that are very considerate beyond all care and concern.
7. Conclusion
According to data analysis, three generative artworks led participants to have different feelings, which were timed from their eye-tracking data and verbal responses. Participants, in a net economic way, marked down arousal, pleasant emotions, and cognitive and objective states. Reflective and contemplative experiences were also recorded. Dynamic transitions processed by visual effect systems and attraction effects induced positive emotional responses in viewers; uninfluential effects, indifferent emotional feelings, and different emotional reactions were found in the processing of jittered puppet cells or the computational morphing of grids. These all heightened one’s sense of ambiguity or complexity before their eyes. The results presented in this paper provide helpful insight into what emotions generative art may evoke, shedding light on how generational features can produce a particular kind of feedback. Findings of this nature are essential for generative art projects targeting digital media, user interface design or advertising. With careful planning, designers can steer audiences to an emotional state by discerning which aspects of an artwork may cause many different impressions. The potential uses of generative art are broader than those commonly practised — for example, in therapeutic settings, where deep calm may be fostered, or narrative and participation, where it may foster feelings of awareness, comfort and good emotional health. By considering their emotional reactions, designers may cater generative art experiences to audiences’ tastes and requirements. Combined with the increasing abilities of generative systems, this provides an exciting prospect to create emotionally resonant and captivating encounters. This is the contribution of this investigation to artists, designers, and scholars alike in the pursuit of emotionally animated and captivating experiences. It indicates that this study requires further elaboration, and thus is felt to be an initial exploration. Although people have been using ‘generative art’ rather loosely for some time, little empirical research has been done into how people feel about generative art. It is thus advisable to regard this as a preliminary investigation and wait for more information to become available. The researchers should continue their work into the generative art-emotional response relationship, drawing on the insights and limitations noted earlier in this initial study.
Notes
Copyright : This is an Open Access article distributed under the terms of the Creative Commons Attribution Non-Commercial License (http://creativecommons.org/licenses/by-nc/3.0/), which permits unrestricted educational and non-commercial use, provided the original work is properly cited.
References
- Alvarez-Melis, D., & Amores, J. (2017). The emotional GAN: Priming adversarial generation of art with emotion. 2017 NeurIPS Machine Learning for Creativity and Design Workshop.
-
Barringer, T. (2016). Art, Music, and the Emotions in the Aesthetic Movement. 19: Interdisciplinary Studies in the Long Nineteenth Century, 2016(23), 1-32.
[https://doi.org/10.16995/ntn.784.]
-
Boden, M. A., & Edmonds, E. A. (2019). From fingers to digits: An artificial aesthetic. MIT Press.
[https://doi.org/10.7551/mitpress/8817.001.0001]
-
Cheung, M.-C., Law, D., Yip, J., & Wong, C. W. (2019). Emotional responses to visual art and commercial stimuli: Implications for creativity and aesthetics. Frontiers in Psychology, 10(14), 415000.
[https://doi.org/10.3389/fpsyg.2019.00014]
-
Else, J. E., Ellis, J., & Orme, E. (2015). Art expertise modulates the emotional response to modern art, especially abstract: An ERP investigation. Frontiers in Human Neuroscience, 9(525), 1-18.
[https://doi.org/10.3389/fnhum.2015.00525]
-
Finisguerra, A., Ticini, L. F., Kirsch, L. P., Cross, E. S., Kotz, S. A., & Urgesi, C. (2021). Dissociating embodiment and emotional reactivity in motor responses to artworks. Cognition, 2021-07(212), 104663-104663.
[https://doi.org/10.1016/j.cognition.2021.104663]
- Jha, D., Chang, H., & Elhoseiny, M. (2021). Wölfflin's Affective Generative Analysis for Visual Art. Proceedings of the 12th International Conference on Computational Creativity (ICCC' 21) (pp. 429-433).
-
Juslin, P. N., & Västfjäll, D. (2008). Emotional responses to music: The need to consider underlying mechanisms. Behavioural and Brain Sciences, 31(5), 559-575.
[https://doi.org/10.1017/S0140525X08005293]
-
Kesner, L., & Horáček, J. (2017). Empathy-related responses to depicted people in art works. Frontiers in Psychology, 8, 228-228.
[https://doi.org/10.3389/fpsyg.2017.00228]
- Lee, Y. K., Park, Y.-H., & Hahn, S. (2023). A portrait of emotion: Empowering self-expression through AI-generated art. arXiv preprint arXiv:2304.13324, 1-7.
- Lukichev, R. V., Skljadnev, M. K., & Chervakov, K. S. (2019). The study of the deformability and strength of polymer geomembranes under uniaxial tension. In Days of Student Science: collection of reports of a scientific and technical conference based on the results of research work by students of the Institute of Hydrotechnical and Power Engineering (pp. 65-70).
-
Matravers, D. (1991). Art and the feelings and emotions. The British Journal of Aesthetics, 31(4), 322-331.
[https://doi.org/10.1093/bjaesthetics/31.4.322]
-
Miu, A. C., Pițur, S., & Szentágotai-Tătar, A. (2016). Aesthetic emotions across arts: A comparison between painting and music. Frontiers in Psychology, 2016-01(6), 1951-1951.
[https://doi.org/10.3389/fpsyg.2015.01951]
- Rank, O. (1932). Art and artist. Knopf.
-
Ristić, I. J., & Milošević, M. (2022). Emotions and familiarity of content in generative processes of prospective artists. Studia Psychologica, 64(4), 356-370.
[https://doi.org/10.31577/sp.2022.04.859]
-
Rotenberg, C. T. (1985). A psychoanalytic study of the appreciation of art. Journal of the American Academy of Psychoanalysis, 13(1), 113-127.
[https://doi.org/10.1521/jaap.1.1985.13.1.113]
-
Spaid, S. (2021). Value Disgust: Appreciating Stench's Role in Attention, Retention and Deception. Rivista di estetica 78(78), 74-94.
[https://doi.org/10.4000/estetica.8586]
-
Sullivan, E., & Herzfeld-Schild, M. L. (2018). Introduction: Emotion, history and the arts. Cultural History, 7(2), 117-128.
[https://doi.org/10.3366/cult.2018.0169]
- Suneson, T. (2023). Memory for Metaphors: Verbatim memory held for literal sentences vs. metaphors. Psychological Science Undergraduate Honors Theses Retrieved from https://scholarworks.uark.edu/psycuht/39.
-
Thagard, P. (2019). Brain-mind: From neurons to consciousness and creativity (Treatise on mind and society). Oxford University Press.
[https://doi.org/10.1093/oso/9780190678715.001.0001]
-
Van Paasschen, J., Zamboni, E., Bacci, F., & Melcher, D. (2014). Consistent emotions elicited by low-level visual features in abstract art. Art & Perception, 2(1-2), 99-118.
[https://doi.org/10.1163/22134913-00002012]