
Understanding Fashion Designers’ Behavior Using Generative AI for Early-Stage Concept Ideation and Revision
Abstract
Background Generative AI has emerged as a powerful tool in fashion design, supporting activities such as ideation, sketching, and creativity enhancement. This study explores how commercial generative AI tools like Bing, Midjourney, and DALL-E can augment the creative processes of professional fashion designers during the early stages of concept ideation and revision.
Methods A mixed-methods approach was employed, involving a pilot study, sketch experiment interviews, and a focus group discussion. 19 participants, including design students and professionals, used AI tools Midjourney and DALL-E for concept ideation and revision. Data were analyzed using thematic analysis, focusing on understanding designers’ behavior and decision-making processes while using generative AI tools.
Results Generative AI tools significantly enhanced the early stages of fashion design by providing diverse and rapid visual stimuli. Designers interacted with AI tools through text prompts, refining hand-drawn sketches, and enhancing digital sketches, which facilitated creativity and decision-making. Challenges identified included biases in AI outputs, intellectual property concerns, and usability issues.
Conclusions The study highlights the potential of generative AI to enhance the fashion design process by providing diverse visual stimuli and accelerating concept ideation and revision. The study identifies key challenges such as biases, intellectual property concerns, and gaps between designer intentions and AI realizations. Future research should explore a broader range of AI tools, long-term impacts, and ethical implications, underscoring AI’s role in augmenting and co-creating, rather than replacing, human creativity in fashion design.
Keywords:
Generative AI, Fashion, Design, Human-AI Co-Creation, Concept Design1. Introduction
The integration of intelligent tools into design processes is becoming increasingly common, supporting activities such as ideation, sketching, and fostering creativity (Tholander & Jonsson, 2023). Tools such as ChatGPT1) enable natural language interactions with AI, significantly impacting various creative domains (Kirmani, 2022). Text-to-image generators like DALL-E2), Midjourney3), and Stable Diffusion4) have revolutionized the creation of realistic images and artworks from textual descriptions, with applications in fields such as poetry, music, animation, and especially fashion design (Gero & Chilton, 2019; Huang et al., 2020; Jeon et al., 2021; Mazzone & Elgammal, 2019). These tools democratize access to high-quality creative content generation (Lawton & Ibarrola, 2023; Louie et al., 2020) and streamline the creative process for professionals (Desai et al., 2019; Grabe & Zhu, 2023; Shin et al., 2023; Tholander & Jonsson, 2023; Verheijden & Funk, 2023).
With the recent advancement, generative AI’s role in the fashion industry has gained momentum, focusing on improving design processes. Applications include Generative Adversarial Networks (GANs) for apparel design (Cui et al., 2018; Kato et al., 2019) and AI systems like DeepVogue by DeepBlue Technology (Jain, 2019), which use deep generative modeling to support creative efforts (Davis et al., 2023). Recent advancements such as CrossGAI (Deng et al., 2024) and the work by Yan et al. (2022) have developed tools for AI-enhanced sketching and rendering.
Despite advancements in recommendation systems and the generation of fashion images, there remains a knowledge gap regarding how commercial generative AI tools like Bing5), Midjourney, and DALL-E can be integrated into traditional fashion design processes to augment and accelerate creativity. Understanding these gaps is crucial for designing effective creativity support tools that enhance human creativity in the early stages of design ideation and revision. For instance, the extensive process of image collection for inspiration involves shopping trips, catwalk visits, trade shows, and desk research (Mete, 2006). AI’s core function of image generation is well-suited to support the transition from concept development to design development, as shown in Figure 1.
Considering the traditional process, there are three key knowledge gaps in applying current generative AI tools to the early stages of the fashion design process. First, user behavior and requirements: Fashion designers have unique needs that influence how they collect and create inspiration. Without a clear understanding of these needs, it is challenging to design user-friendly (Oppenlaender, 2022) and effective AI systems (Zamfirescu et al., 2023). Research should identify the specific outputs designers find useful, the control they need over AI, and the most helpful types of feedback. Second, understanding designer-AI interaction: Limited research exists on how professional fashion designers interact with generative AI tools. Understanding how designers use these tools to generate and refine concepts (Oppenlaender, 2022), the challenges they face, and how they incorporate AI outputs into their decision-making is essential for tailoring AI tools to fit seamlessly into the design process. Third, impact on creativity and workflow: The impact of generative AI on the creativity and workflow of fashion designers is not well understood. Research is needed to explore how AI tools influence the creative process, from idea generation to concept refinement (Zamfirescu et al., 2023), and whether they enhance or limit creativity by steering designers toward specific solutions. Additionally, ethical and practical concerns, such as authorship, intellectual property, data privacy, and bias in AI-generated designs, are critical for broader AI tool acceptance in fashion design. Addressing these concerns is essential for integrating AI responsibly and effectively.
This study aims to bridge these gaps by exploring how fashion designers use generative AI for early-stage concept ideation and revision, focusing on tools like Bing, Midjourney, and DALL-E. Through detailed interviews and experiments with fashion experts, the research provides empirical insights into how these tools support and augment the creative processes of professional fashion designers, highlighting the benefits, challenges, and ethical considerations associated with AI in fashion design.
The research addresses three key questions:
- 1) How does generative AI impact the creativity of fashion designers during the early stage of the design process for ideation and revision?
- 2) What are the interactions between human designers and AI-generated sketches, and how do these interactions influence design decisions?
- 3) What ethical issues and biases arise from the use of generative AI in fashion design, and how can they be mitigated?
To explore these questions, we used a mixed-methods approach that included a pilot study, sketch experiment interviews, and a focus group discussion. 19 participants, including design students for the pilot study and professionals, used the AI tools Midjourney and DALL-E to generate fashion sketches for data collection. Data were analyzed using thematic analysis, focusing on the evaluation of AI-generated sketches and the decision-making processes of professional designers.
By integrating interviews and experiments with fashion experts, this study provides a rare exploration of AI-generated design in the fashion industry. The findings provide valuable recommendations on how to better utilize AI as a tool for fashion designers, how to integrate AI-based design tools and workflows, and how to develop AI-based fashion platforms for future applications, especially in the early stages of the design process. This research represents a pioneering effort to understand the intersection of AI and fashion design, providing a comprehensive analysis that benefits both academia and industry.
2. Related Work
By understanding the nuances of fashion design processes, the role of inspiration, the opportunities and challenges of using current AI applications, and ethical considerations, we can design a user study that effectively explores how generative AI impacts creativity, designer interactions, and ethical issues in the early stages of fashion design. These insights ensured that our research is grounded in existing knowledge while pushing the boundaries to develop more effective and responsible AI tools for the fashion design process.
2. 1. The Process of Fashion Design
The fashion design process includes various stages, from trend research and initial concept development to final product readiness for the market (Harreis et al., 2023) as shown in Figure 1. Researchers have identified different models, such as the seven-stage model: accept, analyze, define, ideate, select, implement, and evaluate (Jeon et al., 2021; Koberg & Bagnall, 1974; Munasinghe et al., 2021; Watkins, 1988), and a six-step model: trend research, trend pack development, design development, prototype development, finalization of range, and approval (Fung & Choi, 2018). This process can be condensed into three main phases: idea generation, product development, and production (ElShishtawy et al., 2022; LaBat & Sokolowski, 1999; Kincade et al., 2007). Understanding these steps helped us explore how generative AI tools can be integrated at various stages, particularly during ideation and concept development, to enhance creativity and efficiency.
2. 2. Generative AI in Use for Fashion Idea Generation
Idea generation in fashion design involves collecting and synthesizing inspiration to create new design concepts, a critical process for fostering creativity and innovation (Plucker et al., 2004). Creativity in fashion design is influenced by factors like visual thinking and the continuous search for inspiration from diverse sources such as nature, artwork, history, music, and technology (Mete, 2006; McKim, 1980). Traditional tools like sketches, collages, and mock-ups help transform tacit knowledge into tangible designs designs (Sarmakari & Vanska, 2022; Keller, 2005). While AI can assist in discovering inspirational stimuli, it may also lead to design fixation and inhibit divergent thinking (Wadinambiarachchi et al., 2024; Cai et al., 2023). These insights informed our exploration of how generative AI tools impact design activities, assessing whether they enhance or hinder creative thinking, as Kwon et al. (2023) noted, and how they influence interactions between designers and AI-generated sketches.
Recent research on AI in fashion design has focused on enhancing creativity, personalizing fashion recommendations, optimizing supply chain management, and improving sustainability (Bhattacharya et al., 2022; Dong et al., 2019; Hidayati et al., 2018; Hou et al., 2021). Advanced tools like DMGAN, CLIP, VQGAN+CLIP, and CLIP-guided GAN have proven beneficial in accelerating the design process by providing detailed and diverse design options quickly (Zhu et al., 2019; Radford et al., 2021; Crowson et al., 2022; Smith & Colton, 2021). These tools enable designers to explore various possibilities with fewer resources, representing a new approach to design thinking (Lee & Lin, 2023). Understanding these capabilities and limitations helped us investigate how AI impacts designers’ decision-making processes and interactions with AI-generated outputs, providing a benchmark for evaluating the effectiveness of AI tools.
2. 3. Potential Limitations of Generative AI for Fashion Design
Generative AI faces several ethical and technical challenges, such as bias in training data, intellectual property concerns, and the tendency to produce designs reflecting existing dominant styles (Flick & Worrall, 2022). For example, the fashion label Acne Studios’ approach of incorporating GAN generations into their Fall 2020 Menswear Collection (Helen, 2020). Also, these tools could inadvertently marginalize minority groups (Flick & Worrall, 2022; Lawton et al., 2023) and lack the novelty needed for truly innovative designs (Grabe & Zhu, 2023). These tools can inadvertently marginalize minority groups and lack the novelty needed for truly innovative designs (Grabe & Zhu, 2023). Also, it is suggested that AI-generated designs often overlook the specific needs of minority groups, including designs for larger individuals, people with disabilities, and those with atypical body shapes (Flick & Worrall, 2022). The subjective and intuitive nature of inspiration in fashion design poses additional challenges for AI integration (Mete, 2006; Stappers & Hennessey, 1999). Understanding these limitations is essential for addressing the ethical issues and biases arising from the use of generative AI in fashion design. Identifying these challenges helped us develop strategies to ensure responsible and inclusive use of AI, directly addressing the third research question on ethical issues and biases.
3. Methods
3. 1. Pilot Study
Prior to the main study, a pilot study was conducted to test the experimental design and ensure the feasibility of the proposed methods. Seven design students from the (UNIST) participated in this preliminary phase. The pilot study involved a simplified version of the main experiment, where participants engaged with AI tools to create fashion sketches. Feedback from this pilot informed refinements to the experimental design, ensuring that the tasks were clear and that the tools used were appropriate for eliciting meaningful data. We built on our research methods from (Gmeiner et al., 2023; Yildirim et al., 2022; Koch et al., 2019) that they conducted a similar research experiment, but in product design and UX/UI design topic. We tailored the the experiment into fashion design filed by modifying the design task into a fashion topic.
3. 2. Participant Recruitment
Participants for the main study were recruited using a snowball sampling method, ensuring a diverse range of fashion design professionals. Initial contacts were made through a known fashion designer, who then recommended additional participants. This method was chosen to include designers with varying levels of experience and expertise, ensuring a comprehensive understanding of how different professionals interact with AI tools. In total, 19 participants were recruited, comprising a mix of students, industry professionals, and researchers in fashion design. Demographic information of the participants are presented in Tables 1 and 2 respectively.
3. 3. Experimental Tools
The primary AI tools used in the study were Midjourney and DALL-E, both of which are text-to-image generators capable of producing high-quality fashion sketches from textual descriptions. The choice of tools was influenced by feedback from the pilot study, where participants preferred Midjourney for its user-friendliness and superior output quality compared to other tools like Stable Diffusion. Each participant was provided with access to these tools during the experiment, ensuring consistency in the AI-generated outputs.
3. 4. Main Study Designs
The main study consisted of two components: (1) sketch experiment interviews and an (2) expert focus group discussion.
(1) Sketch Experiment Interviews
Eight fashion designers participated in the sketch experiment interviews, conducted via Zoom. The sessions were structured into three phases:
- • Warm-Up Exercises (10 minutes): Participants engaged in basic tasks to familiarize themselves with the AI tools. This phase aimed to reduce any initial hesitation and ensure participants were comfortable using the technology.
- • AI Sketches Drawing Session (5-40 minutes): Participants were tasked with creating one to two fashion sketches for their company’s new season development using the AI tools. They were encouraged to base their prompts on recent real projects, making the experiment more realistic and relevant to their professional work. Participants were allowed to choose, revise, and iterate on their prompts independently, simulating a quasi-real design environment.
- • In-Depth Interviews (20 minutes): After the sketching session, participants engaged in a detailed interview where they discussed their experiences using the AI tools. These interviews were recorded and transcribed for further analysis. Participants were asked to evaluate the AI-generated sketches using a five-point rating scale across five criteria: Visual Fidelity and Realism, Originality and Creativity, Aesthetics and Composition, Practicality and Wearability, and Designer Input and Customization.
(2) Expert Focus Group Discussion
A two-hour online focus group discussion was conducted with 11 fashion practitioners. This session aimed to explore the broader implications of AI-generated designs in the fashion industry. Topics included the strengths and weaknesses of AI in creative processes, the potential impact on traditional design practices, and the opportunities AI presents for future design work. The focus group provided a platform for participants to share their diverse perspectives and experiences, enriching the study’s findings.
3. 5. Data Analysis
The data collected from the sketch experiment interviews and the focus group discussion were analyzed using thematic analysis. This method involves coding the data to identify recurring themes and patterns, providing a structured approach to understanding the qualitative insights gathered from participants as shown in Figure 2. The coding process was facilitated by ATLAS.ti, ensuring systematic and rigorous analysis through triangulation.
3. 6. Evaluation Criteria
The evaluation of AI-generated sketches was based on a six-question form, focusing on multiple criteria to assess the quality, creativity, and practicality of the designs based on (Lupton, E., & Phillips, J. C., 2015), (Leborg, C., 2006), and (Culpepper, M. K., 2013). We interpret and revise the categories to fit the fashion design field. The criteria were:
- • Visual Fidelity and Realism: Assessing the accuracy and detail of the AI-generated images.
- • Originality and Creativity: Evaluating the uniqueness and innovative aspects of the designs.
- • Aesthetics and Composition: Considering the overall visual appeal and layout of the sketches.
- • Practicality and Wearability: Determining the feasibility of producing and wearing the designs.
- • Designer Input and Customization: Measuring the extent to which designers could influence and personalize the AI-generated outputs.
Participants rated these criteria on a five-point scale and provided qualitative feedback, highlighting the strengths and limitations of the AI tools in their creative processes.
As for the visualization materials, we output all the AI-generated images and their prompts from the sketch experiments and uploaded them to Figma. We then organized them by participant and by time, and analyzed each participant’s iteration process as shown in Figure 3. This figure represents a workflow map of participant S6, who uses Midjourney as a tool during his design process. These results could provide a comprehensive understanding of the generative design tools in enhancing the ideation process and creating new meanings and experiences for fashion designers.
3. 7. Ethical Considerations
We obtained approval from the Institutional Review Board (IRB) at our institution to ensure that all aspects of our experiment met ethical standards. All participants gave informed consent. Their confidentiality and privacy were strictly maintained throughout the study.
4. Results
The results of this study provide critical insights into how generative AI tools can support and augment the creative processes in the early stages of fashion design, the nature of designers’ interactions with AI-generated sketches, and the challenges and ethical considerations associated with using these tools.
4. 1. The designers’ evaluation of AI-generated fashion sketches
Participants rated AI-generated fashion sketches based on Visual Fidelity and Realism, Originality and Creativity, Aesthetics and Composition, Practicality and Wearability, and Designer Input and Customization. Designers rated Visual Fidelity and Realism the highest, while Designer Input and Customization received the lowest scores as shown in Table 3. However, designers considered Designer Input and Customization to be the most important category, highlighting a significant gap between AI capabilities and designer needs. Originality and creativity, while considered critical, were also areas where AI performed poorly. These findings suggest that future AI development should focus on improving customization and creativity to better meet the needs of fashion designers.
4. 2. Human designers and AI-generated tools interaction in creative decision-making
Fashion designers utilized AI tools in three primary modes:
Text-to-Sketch for Rapid Concept Realization: Designers use text prompts to generate initial sketches when they have vague concepts in mind as shown in Figure 4. This method provides a tangible representation of their ideas, boosting confidence (Participant S6).
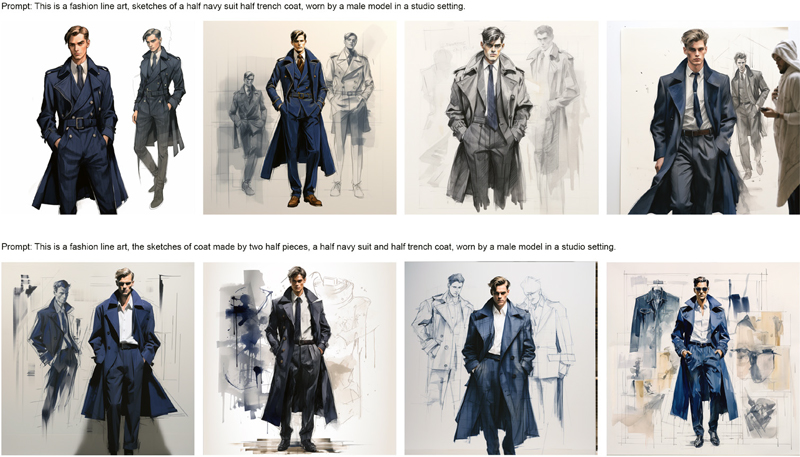
AI-generated fashion sketches by S6 using Prompt 1: This is a fashion line art, sketches of a half navy suit half trench coat, worn by a male model in a studio setting; Prompt 2: This is a fashion line art, the sketches of coat made by two half pieces, a half navy suit and half trench coat, worn by a male model in a studio setting.
Hand-Drawn Sketch Enhancement: Designers input hand-drawn sketches into AI tools to add more detailed designs as showan in Figure 5. This is particularly helpful for those with weaker digital sketching skills (Participant S3).
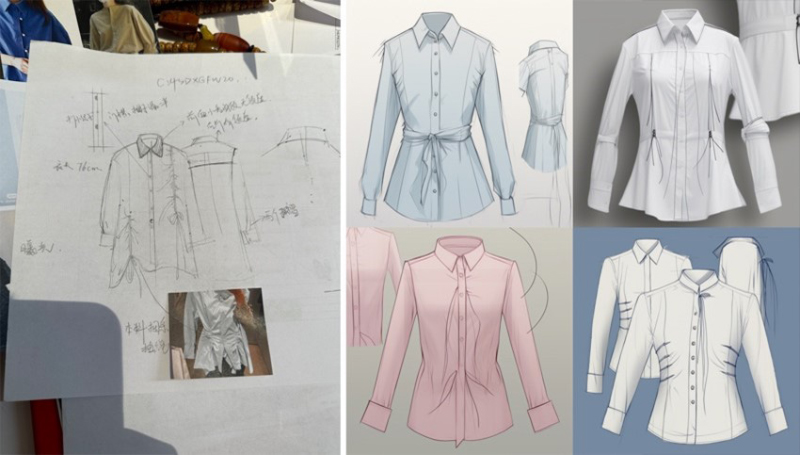
AI-generated fashion sketches by S3. Left: The original fashion design hand drawing sketch input; Right: AI-generated design variation using Prompt: Please design a lady's sun protection shirt with princess line drawstring pleats on the front piece. This shirt can be completely folded and stowed in the left pocket. Please show how the stowage is done and how it is made. Clothing design style, effect.
Software-Assisted Sketch Enhancement: Designers use AI to enhance sketches created with computer software like Adobe Illustrator as shown Figure 6, aiming to visualize fabrics and techniques realistically without creating 3D simulation samples (Participant S5).
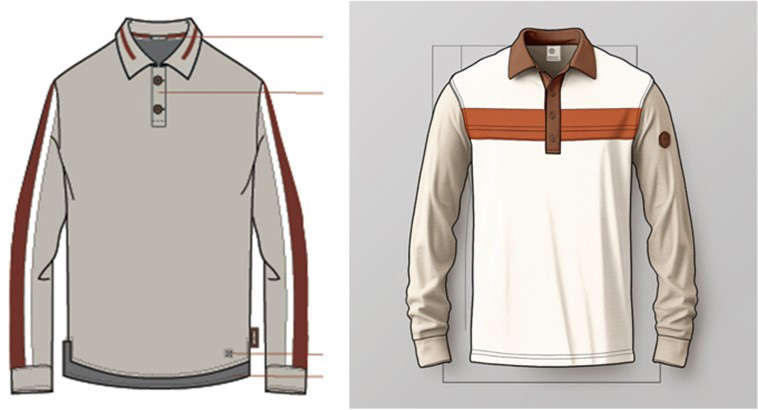
Left: The original fashion design digital drawing input by S5; Right: AI-generated design variation using Prompt: render this knitwear shirt, causal look, design for men -iw 2.
These modes highlight the diverse ways in which AI tools can be integrated into the design process, enhancing different aspects of creative decision-making. Understanding these interactions helps tailor AI tools to better support designers’ workflows.
Some participants emphasized that AI-generated images can provide inspiration and new directions when creativity or artistic skills are lacking. They suggested that AI tools can provide inspiration and new directions when designers face creative blocks. These tools can help visualize and expand conceptual ideas, which can help with brand planning and visual aesthetics (Zhang et al., 2023). They also suggested that AI can combine knowledge from different fields, generating unique design ideas that go beyond individual inspiration. This interdisciplinary approach enriches the creative process, as seen in designs that combine Issey Miyake’s style with other elements (Hwang, 2022; Participant F2). AI’s ability to serve as a bridge and blender underscores its role in enhancing creativity and helping designers explore different styles and concepts.
Designers iteratively refine AI-generated images by modifying prompts to align outputs with their vision. Experienced users create more accurate prompts, resulting in outputs that closely match their intentions (Participants S1, S2, S4, S5, S7). However, a richer experience did not necessarily translate into faster task completion (Figure 7). AI provides designers with endless ideas and experimentation (Figure 8). However, S5 also noted that if designers do not have a firm grasp of the intended topic and rely too heavily on AI, they risk being influenced by AI and deviating from their original design intent. Therefore, the iterative nature of using AI tools reflects their potential to enhance design accuracy and creativity. The need for clear design concepts and frameworks remains critical to prevent over-reliance on AI and ensure human-centered design outcomes, as suggested by Liu (2023).
4. 3. Challenges, biases, and ethical considerations in fashion design
Participants expressed concerns that AI might inhibit deep thinking by providing straightforward design outcomes lacking reflective dialogue between designers and their media (Maher & Poon, 1996; Schon, 1983). AI-generated images are often perceived as less valuable than designers’ original ideas (Participant S5). There is also a lack of comprehensive copyright laws addressing ownership of AI-generated materials.
AI-generated designs can exhibit randomness, leading to misalignment with designers’ intentions (Verheijden & Funk, 2023). Translating ideas into AI prompts often results in less rich or accurate images due to the characteristics of generative AI’s image synthesis mechanism. Enhancing designers’ knowledge of AI prompts and improving AI’s understanding of design terminology is critical for better alignment between AI outputs and creative visions as Figure 9 illustrated. These challenges emphasize the importance of building designers’ knowledge of AI prompts and descriptive logic to improve alignment between AI outputs and their creative visions. Enhancing AI’s understanding of design terminology is also critical.
AI-generated designs can exhibit randomness, leading to misalignment with designers’ intentions. The complexity of translating ideas into AI prompts often results in less rich or accurate images (Verheijden & Funk, 2023). Misinterpretation of fashion design terminology further complicates the process (Participant S5). Participants believed that AI had a limited understanding of some very novel ideas and unique styles, or ideas that did not follow the existing logic. An example is from S6“We found that the AI didn’t understand the concept fully and generated mostly images of people wearing traditional coats. We attempted to provide a similar image to guide its generation, but the result still didn’t match our mental image... it operates based on existing content and doesn’t conform to uncommon design principles”. AI also have a limited understanding of design principles and human aesthetics. For instance, S1 mentioned that AI is struggling to grasp the reasonable distribution of muscles and appropriate balance. Moreover, F8 explained: “AI is great for creativity and concepts, but clothing needs physical representation, and this intermediate process depends on many factors”. AI has limitations in comprehending unconventional or highly original ideas that defy standard logic. Recognizing these limitations is crucial for developing AI tools that better support the unique and innovative aspects of fashion design. AI should be seen as a complementary tool rather than a replacement for human creativity and detailed craftsmanship.
4. 4. Impact on transforming the fashion design process with generative AI
Participants highlighted the role of generative AI in enhancing efficiency and inspiring creativity during the initial stages of design as Tholander & Jonsson (2023) and Verheijden & Funk (2023) suggested. AI-generated designs are better suited for inspiration rather than final products, aiding in brand planning and image searches but requiring human intervention for execution and detailed design (Participants S1, S2, S4); this confirms (Tholander & Jonsson, 2023; Zhang et al., 2023)’s results. These insights suggest that AI tools should be viewed as starting points for creativity, complemented by human expertise for practical implementation.
Participants identified a gap in the market for AI generative design platforms specifically tailored to fashion design. While platforms like AiDLab6), Fabrie127), and Zhiyi Tech8)’s Fashion Diffusion Beta exist, they do not fully meet the specific needs of clothing design. Developing specialized AI tools with tailored functionalities, detailed instructions, and advanced search capabilities can better support designers’ creative processes as Chiou et al. (2023) and Yildirim et al. (2022) noted.
The integration of AI in fashion design is creating new roles and responsibilities for designers. However, there is a disparity between the demand for AI skills and the availability of training and real project opportunities. To bridge this gap, fashion companies need to provide more structured training and project opportunities for designers to develop AI skills. Fashion education programs should also incorporate AI training to prepare future designers for effective collaboration with these technologies (Participants S3, S4, F7).
5. Discussion
The results of this study provide substantial insights into the role and impact of generative AI tools in the early stages of fashion design, highlighting both the potential benefits and the challenges of existing tools. The findings suggest the importance of customization, interaction dynamics, and ethical considerations for the future creative workflow of fashion designers.
5. 1. Impact of generative AI on the early design activities in Fashion Design
The evaluation of AI-generated fashion sketches reveals that while AI excels in visual fidelity and realism, it falls short in originality, creativity, and customization. This disparity points to a crucial area for improvement in AI tools. For AI to genuinely augment the creative process, it must offer greater flexibility and customization options that align more closely with designers’ needs. The true value of AI lies in its capacity to inspire and foster unique, original ideas. Future AI development should prioritize enhancing creative functionalities and providing more sophisticated customization features to support the ideation phase more effectively.
5. 2. Interactions between designers and AI-generated sketches
The study highlights diverse modes of interaction between human designers and AI tools, including text-to-sketch generation, hand-drawn sketch enhancement, and software-assisted sketch refinement. These interactions illustrate how AI can be integrated into different stages of the design process, enhancing creativity and decision-making. Using text prompts to quickly visualize vague concepts boosts designers’ confidence, while enhancing hand-drawn sketches with AI helps refine ideas more efficiently. AI tools act as bridges, providing inspiration and new directions when designers face creative blocks. This cross-disciplinary blending of ideas enriches the creative process by enabling designers to explore a broader range of styles and concepts. However, the iterative use of AI, where designers refine outputs through repeated prompt modifications, highlights a potential dependency risk. While AI can offer endless ideas and experimentation opportunities, there is a risk of designers deviating from their original intent if they overly rely on AI-generated suggestions. Designers need to maintain a clear framework and design concept to ensure AI complements rather than overshadows their creative vision.
5. 3. Ethical issues in data and biases in visual materials generated
The study identifies several ethical concerns and biases associated with AI-generated designs. Participants expressed worries about AI inhibiting deep, reflective thinking, as the straightforward design outcomes provided by AI can lack the nuanced dialogue between designer and media essential for creativity. The perception that AI-generated images are less valuable than original ideas also highlights a significant challenge in integrating AI into creative processes. Additionally, the lack of comprehensive copyright laws for AI-generated materials poses a legal and ethical dilemma, emphasizing the need for clearer regulations to protect designers’ intellectual property.
Understanding issues with AI, such as the randomness and misalignment of AI-generated designs with designers’ intentions, complicates the integration of AI in fashion design. Translating ideas into AI prompts often results in less rich or accurate images, highlighting the importance of building designers’ knowledge of AI prompts and improving AI’s understanding of design terminology. Enhancing AI’s ability to accurately interpret and generate designs based on specific fashion terminology is critical for better alignment with designers’ creative visions.
5. 4. Practical and ethical implications
To fully leverage the benefits of generative AI in fashion design, developers need to focus on enhancing the customization and creative capabilities of AI tools. Providing designers with more control over AI-generated outputs and integrating advanced customization options will help align these tools with the specific needs of the fashion industry. Ethically, it is crucial to address the biases and limitations of AI-generated designs. Ensuring that AI tools do not perpetuate existing biases or marginalize minority groups is essential for creating inclusive and diverse fashion designs. Developing clearer guidelines and regulations regarding the ownership and copyright of AI-generated designs will help protect designers’ intellectual property and foster a more ethical integration of AI in fashion design.
6. Conclusion
This study provides substantial insights into the role of generative AI tools in the early stages of fashion design, highlighting their potential to enhance creativity and streamline the design process. AI tools like Midjourney, DALL-E, and Bing can significantly augment the creative processes of fashion designers by providing rapid, diverse visual stimuli. However, the findings reveal that while AI excels in generating visually realistic and detailed images, it falls short in originality, creativity, and customization. To genuinely augment the creative process, future AI development must prioritize enhancing these creative functionalities and offering more sophisticated customization options.
Interactions between designers and AI tools are diverse, ranging from text-to-sketch generation to hand-drawn and software-assisted sketch enhancements. These interactions help integrate AI into different stages of the design process, enhancing creativity and decision-making. Nevertheless, the iterative use of AI highlights a potential dependency risk, underscoring the need for designers to maintain a clear framework and design concept to ensure AI complements rather than overshadows their creative vision.
Ethical concerns and biases in AI-generated designs present significant challenges. Issues such as inhibiting deep reflective thinking, perceived lower value of AI-generated images, and the lack of comprehensive copyright laws for AI-generated materials highlight the need for ethical guidelines and clearer regulations. Addressing these concerns is crucial for the responsible and inclusive use of AI in fashion design.
Despite its contributions, this study has several limitations. The relatively small sample size and the specific demographic of participants may limit the generalizability of the findings. The study also primarily utilized Midjourney and DALL-E, which, while effective, represent only a subset of available AI tools, and the design tasks in this study were limited to initial ideation and sketching. Lastly, this study did not address into the long-term impact of generative AI on the fashion design process and desginers’ reliance.
Future research should explore a broader range of AI tools and their long-term impacts on the fashion design process. Investigating how AI can be tailored to support specific design needs and preferences will be crucial. Furthermore, addressing ethical concerns and biases through comprehensive studies will help develop clearer guidelines and regulations for AI integration in fashion design. Expanding the scope to include the later stages of the design process will provide a more holistic understanding of AI’s role in the fashion industry. Finally, future work should consider larger, more diverse sample sizes to validate and extend the findings of this study.
Glossary
1) ChatGPT: https://openai.com/blog/chatgpt/
2) DALL-E: https://openai.com/dall-e-2/
3) Midjourney: https://www.midjourney.com/
4) Stable Diffusion: https://stablediffusionweb.com/
5) Bing: https://www.bing.com/images/create
6) AiDLab: https://www.aidlab.hk/en/
7) Fabrie: https://www.fabrie.com/
8) Zhiyi Tech: https://www.zhiyitech.cn/home
Acknowledgments
This research was partially supported by Korea Institute for Advancement of Technology (KIAT) grant funded by the Korea Government (MOTIE)(P0025495, Establishment of Infra-structure for Integrated Utilization of Design Industry Data). This work was also partially supported by the Technology Innovation Program (20015056, Commercialization design and development of Intelligent Product-Service System for personalized full silver life cycle care) funded by the Ministry of Trade, Industry & Energy (MOTIE, Korea).
Notes
Copyright : This is an Open Access article distributed under the terms of the Creative Commons Attribution Non-Commercial License (http://creativecommons.org/licenses/by-nc/3.0/), which permits unrestricted educational and non-commercial use, provided the original work is properly cited.
References
-
Bhattacharya, G., Kilari, N., Gubbi, J., & Pal, A. (2022). DAtRNet: Disentangling fashion attribute embedding for substitute item retrieval. In Proceedings of the IEEE/CVF Conference on Computer Vision and Pattern Recognition (pp. 2283-2287).
[https://doi.org/10.1109/CVPRW56347.2022.00253]
-
Cai, A., Rick, S. R., Heyman, J. L., Zhang, Y., Filipowicz, A., Hong, M., ... & Malone, T. (2023, November). DesignAID: Using Generative AI and Semantic Diversity for Design Inspiration. In Proceedings of The ACM Collective Intelligence Conference (pp. 1-11).
[https://doi.org/10.1145/3582269.3615596]
-
Chiou, L. Y., Hung, P. K., Liang, R. H., & Wang, C. T. (2023, July). Designing with AI: An Exploration of Co-Ideation with Image Generators. In Proceedings of the 2023 ACM Designing Interactive Systems Conference (pp. 1941-1954).
[https://doi.org/10.1145/3563657.3596001]
-
Crowson, K., Biderman, S., Kornis, D., Stander, D., Hallahan, E., Castricato, L., & Raff, E. (2022, October). Vqgan-clip: Open domain image generation and editing with natural language guidance. In European Conference on Computer Vision (pp. 88-105). Cham: Springer Nature Switzerland.
[https://doi.org/10.1007/978-3-031-19836-6_6]
-
Cui, Y. R., Liu, Q., Gao, C. Y., & Su, Z. (2018, October). FashionGAN: display your fashion design using conditional generative adversarial nets. In Computer Graphics Forum (Vol. 37, No. 7, pp. 109-119).
[https://doi.org/10.1111/cgf.13552]
- Culpepper, M. K. (2013). The Telling Line: The Relationship between Cognitive Style and Fashion Design Sketching.
-
Davis, R. L., Wambsganss, T., Jiang, W., Kim, K. G., Kaser, T., & Dillenbourg, P. (2023, April). Fashioning the Future: Unlocking the Creative Potential of Deep Generative Models for Design Space Exploration. In Extended Abstracts of the 2023 CHI Conference on Human Factors in Computing Systems (pp. 1-9).
[https://doi.org/10.1145/3544549.3585644]
-
Deng, H., Jiang, J., Yu, Z., Ouyang, J., & Wu, D. (2024). CrossGAI: A Cross-Device Generative AI Framework for Collaborative Fashion Design. Proceedings of the ACM on Interactive, Mobile, Wearable and Ubiquitous Technologies, 8(1), 1-27.
[https://doi.org/10.1145/3643542]
-
Desai, R., Anderson, F., Matejka, J., Coros, S., McCann, J., Fitzmaurice, G., & Grossman, T. (2019, May). Geppetto: Enabling semantic design of expressive robot behaviors. In Proceedings of the 2019 CHI Conference on Human Factors in Computing Systems (pp. 1-14).
[https://doi.org/10.1145/3290605.3300599]
-
Dong, X., Song, X., Feng, F., Jing, P., Xu, X. S., & Nie, L. (2019, October). Personalized capsule wardrobe creation with garment and user modeling. In Proceedings of the 27th ACM international conference on multimedia (pp. 302-310).
[https://doi.org/10.1145/3343031.3350905]
-
ElShishtawy, N., Sinha, P., & Bennell, J. A. (2022). A comparative review of zero-waste fashion design thinking and operational research on cutting and packing optimisation. International Journal of Fashion design, technology and education, 15(2), 187-199.
[https://doi.org/10.1080/17543266.2021.1990416]
-
Flick, C., & Worrall, K. (2022). The ethics of creative AI. In The Language of Creative AI: Practices, Aesthetics and Structures (pp. 73-91). Cham: Springer International Publishing.
[https://doi.org/10.1007/978-3-031-10960-7_5]
-
Fung, Y. N., & Choi, T. M. (2018). Product development process of an international luxury fashion Brand: Implications to Hong Kong fashion trading and manufacturing companies. Contemporary Case Studies on Fashion Production, Marketing and Operations, 27-41.
[https://doi.org/10.1007/978-981-10-7007-5_2]
-
Gero, K. I., & Chilton, L. B. (2019, May). Metaphoria: An algorithmic companion for metaphor creation. In Proceedings of the 2019 CHI conference on human factors in computing systems (pp. 1-12).
[https://doi.org/10.1145/3290605.3300526]
-
Gmeiner, F., Yang, H., Yao, L., Holstein, K., & Martelaro, N. (2023, April). Exploring Challenges and Opportunities to Support Designers in Learning to Co-create with AI-based Manufacturing Design Tools. In Proceedings of the 2023 CHI Conference on Human Factors in Computing Systems (pp. 1-20).
[https://doi.org/10.1145/3544548.3580999]
- Grabe, I., & Zhu, J. (2023). Towards Co-Creative Generative Adversarial Networks for Fashion Designers. arXiv preprint arXiv:2304.09477.
- Harreis, H., Koullias, T., Roberts, R., & Te, K. (2023, March 8). Generative AI: Unlocking the future of fashion. McKinsey & Company. https://www.mckinsey.com/industries/retail/our-%20insights/generative-ai-unlocking-the-future-of-fashion.
-
Hidayati, S. C., Hsu, C. C., Chang, Y. T., Hua, K. L., Fu, J., & Cheng, W. H. (2018, October). What dress fits me best? Fashion recommendation on the clothing style for personal body shape. In Proceedings of the 26th ACM international conference on Multimedia (pp. 438-446).
[https://doi.org/10.1145/3240508.3240546]
-
Hou, Y., Vig, E., Donoser, M., & Bazzani, L. (2021). Learning attribute-driven disentangled representations for interactive fashion retrieval. In Proceedings of the IEEE/CVF International conference on computer vision (pp. 12147-12157).
[https://doi.org/10.1109/ICCV48922.2021.01193]
- Huang, C. Z. A., Koops, H. V., Newton-Rex, E., Dinculescu, M., & Cai, C. J. (2020). AI song contest: Human-AI co-creation in songwriting. arXiv preprint arXiv:2010.05388.
-
Hwang, A. H. C. (2022, April). Too late to be creative? AI-empowered tools in creative processes. In CHI Conference on Human Factors in Computing Systems Extended Abstracts (pp. 1-9).
[https://doi.org/10.1145/3491101.3503549]
- Jain, M. (2024, May 10). An AI "Designer" just won Runner-Up in a major fashion design competition. Stories From the Center of China's Youth Culture. https://radii.co/article/an-aidesigner-just-won-runner-up-in-a-major-fashion-design-competition.
-
Jeon, Y., Jin, S., Shih, P. C., & Han, K. (2021, May). FashionQ: an ai-driven creativity support tool for facilitating ideation in fashion design. In Proceedings of the 2021 CHI Conference on Human Factors in Computing Systems (pp. 1-18).
[https://doi.org/10.1145/3411764.3445093]
-
Kato, N., Osone, H., Oomori, K., Ooi, C. W., & Ochiai, Y. (2019, March). Gans-based clothes design: Pattern maker is all you need to design clothing. In Proceedings of the 10th Augmented Human International Conference 2019 (pp. 1-7).
[https://doi.org/10.1145/3311823.3311863]
- Keller, A. I. (2005). For Inspiration Only; Designer interaction with informal collections of visual material.
-
Kincade, D. H., Regan, C., & Gibson, F. Y. (2007). Concurrent engineering for product development in mass customization for the apparel industry. International Journal of Operations & Production Management, 27(6), 627-649.
[https://doi.org/10.1108/01443570710750295]
-
Kirmani, A. R. (2022). Artificial intelligence-enabled science poetry. ACS Energy Letters, 8(1), 574-576.
[https://doi.org/10.1021/acsenergylett.2c02758]
- Koberg, D., & Bagnall, J. (1974). The Universal Traveler, A Soft-Systems Guide to: Creativity, Problem Solving, and the Process of Reaching Goals.[Revised Edition.].
-
Koch, J., Lucero, A., Hegemann, L., & Oulasvirta, A. (2019, May). May AI? Design ideation with cooperative contextual bandits. In Proceedings of the 2019 CHI Conference on Human Factors in Computing Systems (pp. 1-12).
[https://doi.org/10.1145/3290605.3300863]
-
Kwon, E., Rao, V., & Goucher-Lambert, K. (2023). Understanding inspiration: Insights into how designers discover inspirational stimuli using an AI-enabled platform. Design Studies, 88, 101202.
[https://doi.org/10.1016/j.destud.2023.101202]
-
LaBat, K. L., & Sokolowski, S. L. (1999). A three-stage design process applied to an industryuniversity textile product design project. Clothing and Textiles Research Journal, 17(1), 11-20.
[https://doi.org/10.1177/0887302X9901700102]
-
Lawton, T., Grace, K., & Ibarrola, F. J. (2023, July). When is a Tool a Tool? User Perceptions of System Agency in Human-AI Co-Creative Drawing. In Proceedings of the 2023 ACM Designing Interactive Systems Conference (pp. 1978-1996).
[https://doi.org/10.1145/3563657.3595977]
-
Lee, Y. H., & Lin, T. H. (2023, July). The Feasibility Study of AI Image Generator as Shape Convergent Thinking Tool. In International Conference on Human-Computer Interaction (pp. 575-589). Cham: Springer Nature Switzerland.
[https://doi.org/10.1007/978-3-031-35891-3_36]
- Leborg, C. (2006). Visual Grammar: A Design Handbook (Visual Design Book for Designers, Book on Visual Communication). Princeton Architectural Press.
- Liu, S., Ou, X., Qian, R., Wang, W., & Cao, X. (2016). Makeup like a superstar: Deep localized makeup transfer network. arXiv preprint arXiv:1604.07102.
-
Liu, V. (2023, April). Beyond Text-to-Image: Multimodal Prompts to Explore Generative AI. In Extended Abstracts of the 2023 CHI Conference on Human Factors in Computing Systems (pp. 1-6).
[https://doi.org/10.1145/3544549.3577043]
-
Louie, R., Coenen, A., Huang, C. Z., Terry, M., & Cai, C. J. (2020, April). Novice-AI music co-creation via AI-steering tools for deep generative models. In Proceedings of the 2020 CHI conference on human factors in computing systems (pp. 1-13).
[https://doi.org/10.1145/3313831.3376739]
- Lupton, E., & Phillips, J. C. (2015). Graphic design: the new basics (revised and expanded). Chronicle Books.
-
Maher, M. L., & Poon, J. (1996). Modeling design exploration as co-evolution. Computer-Aided Civil and Infrastructure Engineering, 11(3), 195-209.
[https://doi.org/10.1111/j.1467-8667.1996.tb00323.x]
-
Mazzone, M., & Elgammal, A. (2019, February). Art, creativity, and the potential of artificial intelligence. In Arts(Vol. 8, No. 1, p. 26). MDPI.
[https://doi.org/10.3390/arts8010026]
- McKim, R. H. (1980). Thinking visually: A strategy manual for problem solving. Lifetime learning publications.
-
Mete, F. (2006). The creative role of sources of inspiration in clothing design. International journal of clothing science and technology, 18(4), 278-293.
[https://doi.org/10.1108/09556220610668509]
-
Munasinghe, P. D., Dissanayake, D. G. K., & Druckman, A. (2021). An investigation of the mass-market fashion design process. Research Journal of Textile and Apparel, 26(4), 323-342.
[https://doi.org/10.1108/RJTA-08-2020-0089]
-
Oppenlaender, J. (2022, November). The creativity of text-to-image generation. In Proceedings of the 25th International Academic Mindtrek Conference (pp. 192-202).
[https://doi.org/10.1145/3569219.3569352]
- Helen Papagiannis. (2020). ACNE STUDIOS X ROBBIE BARRAT. https://xrgoespop.com/home/acne-studios-x-robbie-barrat.
-
Plucker, J. A., Beghetto, R. A., & Dow, G. T. (2004). Why isn't creativity more important to educational psychologists? Potentials, pitfalls, and future directions in creativity research. Educational psychologist, 39(2), 83-96.
[https://doi.org/10.1207/s15326985ep3902_1]
- Radford, A., Kim, J. W., Hallacy, C., Ramesh, A., Goh, G., Agarwal, S., ... & Sutskever, I. (2021, July). Learning transferable visual models from natural language supervision. In International conference on machine learning (pp. 8748-8763). PMLR.
-
Sarmakari, N., & Vanska, A. (2022). 'Just hit a button!'–fashion 4.0 designers as cyborgs, experimenting and designing with generative algorithms. International Journal of Fashion Design, Technology and Education, 15(2), 211-220.
[https://doi.org/10.1080/17543266.2021.1991005]
- Schon, D. (1983). The Reflective Practitioner (London, Temple Smith). SchonThe Reflective Practitioner1983.
-
Shin, J. G., Koch, J., Lucero, A., Dalsgaard, P., & Mackay, W. E. (2023, April). Integrating AI in Human-Human Collaborative Ideation. In Extended Abstracts of the 2023 CHI Conference on Human Factors in Computing Systems (pp. 1-5).
[https://doi.org/10.1145/3544549.3573802]
- Smith, A., & Colton, S. (2021). Clip-guided gan image generation: An artistic exploration. Evo, 2021, 17.
-
Stappers, P. J., & Hennessey, J. M. (1999). Toward electronic napkins and beermats: Computer support for visual ideation skills. In Visual representations and interpretations (pp. 220-225). London: Springer London.
[https://doi.org/10.1007/978-1-4471-0563-3_24]
-
Tholander, J., & Jonsson, M. (2023, July). Design ideation with ai-sketching, thinking and talking with Generative Machine Learning Models. In Proceedings of the 2023 ACM Designing Interactive Systems Conference (pp. 1930-1940).
[https://doi.org/10.1145/3563657.3596014]
-
Verheijden, M. P., & Funk, M. (2023, April). Collaborative Diffusion: Boosting Designerly Co-Creation with Generative AI. In Extended Abstracts of the 2023 CHI Conference on Human Factors in Computing Systems (pp. 1-8).
[https://doi.org/10.1145/3544549.3585680]
-
Wadinambiarachchi, S., Kelly, R. M., Pareek, S., Zhou, Q., & Velloso, E. (2024). The Effects of Generative AI on Design Fixation and Divergent Thinking. arXiv preprint arXiv:2403.11164.
[https://doi.org/10.1145/3613904.3642919]
-
Watkins, S. M. (1988). Using the design process to teach functional apparel design. Clothing and Textiles Research Journal, 7(1), 10-14.
[https://doi.org/10.1177/0887302X8800700103]
-
Yan, H., Zhang, H., Liu, L., Zhou, D., Xu, X., Zhang, Z., & Yan, S. (2022). Toward intelligent design: An ai-based fashion designer using generative adversarial networks aided by sketch and rendering generators. IEEE Transactions on Multimedia.
[https://doi.org/10.1109/TMM.2022.3146010]
-
Yildirim, N., Kass, A., Tung, T., Upton, C., Costello, D., Giusti, R., ... & Zimmerman, J. (2022, April). How Experienced Designers of Enterprise Applications Engage AI as a Design Material. In Proceedings of the 2022 CHI Conference on Human Factors in Computing Systems (pp. 1-13).
[https://doi.org/10.1145/3491102.3517491]
-
Zamfirescu-Pereira, J. D., Wong, R. Y., Hartmann, B., & Yang, Q. (2023, April). Why Johnny can't prompt: how non-AI experts try (and fail) to design LLM prompts. In Proceedings of the 2023 CHI Conference on Human Factors in Computing Systems (pp. 1-21).
[https://doi.org/10.1145/3544548.3581388]
-
Zhang, C., Wang, W., Pangaro, P., Martelaro, N., & Byrne, D. (2023, June). Generative Image AI Using Design Sketches as input: Opportunities and Challenges. In Proceedings of the 15th Conference on Creativity and Cognition (pp. 254-261).
[https://doi.org/10.1145/3591196.3596820]
-
Zhu, M., Pan, P., Chen, W., & Yang, Y. (2019). Dm-gan: Dynamic memory generative adversarial networks for text-to-image synthesis. In Proceedings of the IEEE/CVF conference on computer vision and pattern recognition (pp. 5802-5810).
[https://doi.org/10.1109/CVPR.2019.00595]